与全球精准医疗共同起航:禽流感范围界定检索
介绍
Avian Influenza也称为“禽流感(Bird Flu)“, 是由将动物种群与人类联系起来的主要病毒之一的病毒引致,预计病死率为 60% [1]。禽流感病毒的遗传物质为单链 RNA[2]。这些病毒可以根据它们引发疾病的严重程度进一步细分为两组,即高致病性禽流感 (HPAI) 和低致病性禽流感 (LPAI)(同上)。每种病毒都包含一个H和一个N抗原,已知H5和H7毒株会引发HPAI。有假设认为HPAI 是 LPAI引发的由有缺陷的聚合酶复合体导致的自发性突变[3]。虽然后者被认为是最易被观察到的病变机制,但研究也发现了其他的替代路径,包括核苷酸替换和与其他基因重组而导致的HPAI 出现(同上)。
禽流感的天然宿主为水鸟,主要通过感染家禽和水禽,以及其他鸟类进行传播。该病毒可以通过粪/口途径传播,也可以通过陆地鸟类的呼吸途径传播[2]。家禽的禽流感爆发尤其令人担忧,因为它有可能从 LPAI 演变为 HPAI,并且 HPAI 造成的家禽死亡与经济损失和贸易限制相关[4]。在动物中,HPAI可能产生鸡群突然出现的高死亡率,与头部和面部水肿、头部皮下出血以及产卵停止等临床症状相关(同上)。然而,最严重的公共卫生问题是禽流感病毒可以传播给人类。疾病或病毒从动物传染给人类(反之亦然)的过程称为人畜共患病[1]。人类感染H5N1型禽流感的首例病例可追溯到 1997年,中国香港共发生了18例确诊病例和6例死亡病例,突显了禽流感大流行的可能性[5]。禽流感也曾发生人传人情况,2015年中国大陆报告了超过200例的H7N9型禽流感病例[6]。动物到人类的传播路径通常是通过受污染的环境或中间宿主而传播,例如猪的屠宰过程中直接接触可能会发生传播[6]。病毒在亚洲具备跨越物种传播的条件,在某些地区,家禽、猪和人类共同生活在狭小的环境中,并且会通过活禽市场发生职业暴露[3]。
为了减少禽流感传播,人们已经采取了多种预防和控制措施。降低动物种群感染风险对降低人类感染风险至关重要[1]。包括世界卫生组织(WHO)、联合国粮食与农业组织(FAO) 和 世界动物卫生组织(OIE) 在内的三方合作制定了相应的指南,用于最常见的禽流感预防和控制[7],指南包括:鸟类种群的疫苗接种、OIE 陆生动物卫生法[8]等法律和生物安全措施。生物安全措施,是指可用于防止禽流感传入易感家禽的物理和/或程序措施[2]。现有的预防和控制策略可以通过数字技术来加强,数字技术可以定义为数字资源,用于从人群中收集新的个人或环境数据(人与动物),数字技术包括但不限于:移动医疗、大数据和遥感技术。例如,时空模型可用于更精确地估计疫情分布,通过卫星遥感技术可以深入了解人类社会及行为模式等。因此,数字技术在加强禽流感的预防和控制工作上展示了巨大的潜力。
研究目的和研究问题
本次范围界定综述的目的是检索关注数字技术和禽流感防控的现有研究,并进一步探索他们在加强禽流感监测的可能。本次范围界定综述旨在回答以下问题:目前有哪些数字技术被用于改进和增强禽流感的检测、控制和预防工作?
研究方法
范围检索旨在“形成知识综述,解决探索性研究问题。通过系统地检索、选择及综合现有研究成果来绘制与特定领域或相关研究中的关键概念、证据类型及差异”[9]。本研究旨在绘制用于应对禽流感的现有数字技术的应用地图。
检索策略
检索策略由三位作者共同制定,包括数字技术和禽流感相关的广泛术语,文本和 MeSH 术语的组合(见附件)。
关键词
用于识别使用数字技术与禽流感防控相关的文献。与疾病相关的关键词(禽流感), 使用来自国家医学图书馆MeSH数据库的MeSH词组及其附属目录同义词进行识别,而与数字技术相关的关键词则通过初步文献筛选的关键词进行识别。再结合疾病相关关键词和数字技术的相关词组,在高级搜索设置中进行筛选(详见附件表S1),例如以下关键词包含但不限于:[(Avian Influenza) 或 (HPAI) 或 (H5N1)] 和 [(Technology) 或 (Big data) 或 (Social media) 或 (mHealth)],用于筛选相关文献。此外,还检索了已筛选出研究的参考文献以收集更多相关资料。
数据库
为了确保对文献进行更全面地检索,综述中包括了两个数据库,即 PubMed 和 Web of Science。利用滚雪球方法和手动检索首先识别出的文献,再从已标记文献数据库中检索出其他的相关文献。
研究的选择、纳入和排除标准
本次综述检索了所有与利用数字技术改善禽流感防控相关结果的研究。本研究也检索了同行评审文献(包括原始的定量和定性研究),以及在 PubMed 和 Web of Science 中编入索引的社论、观点和信件。文献须在 2009 年(一月)和 2017 年(七月)之间以英文、西班牙文、法文或德文发表。对相关研究的地理位置、人种或研究设计没有设限。本次研究排除了重复研究、上述指定出版语言以外的研究,以及以兽医为重点、反对或与公共卫生无关的文献,以及没有明确关于数字技术的研究。
数据的收集和整理
两位作者根据研究标题和摘要与本研究的相关性独立制定收录和排除标准。之后两位作者对筛选出的文献列表合并去重,讨论收录或排除的基本原则,然后从两位审稿人先前制作的两个列表中选择纳入本次综述的选文列表。此外,第三位作者也参与了文献筛选过程,并仔细检索了最终入选的清单。之后获取全文文章并将符合条件的研究总归纳到到描述性总结表中,关注以下关键信息:作者、发表日期、发布期刊、区域、作者单位、数字技术/设备、功能、研究设计、数据源、目标人群、健康指标和挑战。并请留意,数字技术是根据以下指定的研究领域进行分组,按出现频率创建(见表1)。引文使用 EndNote 软件进行管理。
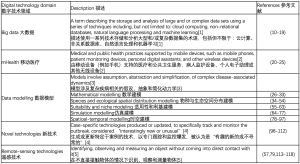
Full table
数据合成
使用文字叙述和图形的组合来合成数据,以对调查结果进行总结性描述。此外,本研究创建了作者国籍的相关性网络,可视化界定学术界中的数字创新研究中心。在作者的国籍关系网络中,每个圆圈的半径反映了每个国家/地区的出版物数量,边缘的颜色取决于它们来自哪个大洲,国家之间的联系代表国家之间的不同合作(见图1)。该图通过在第一作者和其他每个作者之间添加一条连线绘制出的。
结果
主要发现
通过标题和摘要筛共选出1,753篇文章,其中694篇被确认为相关研究,191篇因重复研究被排除,392篇不符合纳入标准,因此最终筛选出 111 项研究纳入综述(见图2)。 综述中纳入的研究确认了用于应对禽流感防控的数字技术或设备。检索的 111 项研究主要围绕五个数字技术领域,即大数据、移动医疗、数据建模、新技术和遥感技术(见表1)。大多数研究发表于 2016 年,占总出版研究数的21%。亚洲的相关研究最多(57%),而南美洲则没有符合纳入标准的已发表文献。
使用作者单位隶属网络对通过以国家/地区划分的现有研究成果进行了可视化(见图1)。最多相关研究的是美国,与中国和比利时有着密切联系。其他主要贡献者位于欧洲,比如比利时、法国、意大利和英国。许多文献引用了比利时国家科学研究基金和布鲁塞尔大学的生物控制和空间生态学部门,以及粮农组织、意大利动物卫生服务机构的EMPRES野生动物部门资源,这可能解释了比利时和意大利作出的的较大贡献 。所选文章中的许多作者所在的国家/地区都隶属于亚洲国家的机构,例如越南、印度、韩国、孟加拉国、日本和柬埔寨,而这些国家正是受禽流感影响较为严重的国家。
数据建模
数据建模占综述中研究的65%,涵盖从计算机辅助数学建模到时空建模(见图1,图3)。数学建模包括基于蒙特卡罗模拟、贝叶斯概率和物种分布模型的研究,主要用于估计禽流感爆发分布、预测宿主一病毒相互作用,以及更准确地研究通过各种场景(含活禽市场)的传播和控制动态。此外,通过物种生态位建模和使用气象数据集来预测和绘制疾病发生概率高的区域,模型产生了更多的生态焦点。
新技术
综述中发现新技术占总文献筛选结果的13%,包括特定病例的诊断设备 (67%) 纳米技术 (26%) 和可穿戴设备 (7%)。新技术主要用于监测目的,而其中一项值得关注的研究是利用纳米技术进行治疗。
大数据
本次综述选出了十项 (10%) 专注于大数据领域的研究,这些研究可以进一步细分为社交媒体分析 (40%)、基于网络的监控平台 (40%) 和在线学习资源 (20%)。社交媒体平台被用来以衡量用户参与度和健康传播活动来捕捉和告知用户的行为变化。大数据平台也被用于以网络监控的形式收集信息,同时也支持在线学习。
遥感技术
少数研究探索了遥感技术(7%),在卫星遥测和卫星图像的参数下,捕捉候鸟种群如何与其处的环境相互作用,并通过地球卫星观测识别受污染的水体。
移动医疗
一小部分研究致力于移动医疗领域,占本次检索结果的5%。移动医疗主要用作监控系统的一部分,通过短信(SMS)或呼叫功能发出报告,以及GPS技术来追踪医护人员和病例。同时,手机等移动设备也被用于诊断目的。
本次检索中认定的数字技术主要用于疫情监控 (83%),部分专门用于诊断(16%),而较少应用于治疗 (1%)。 在监控职能中,数据建模仍占据主导地位,而诊断目的主要依赖新技术和移动医疗(见表2)。虽然大数据相关研究数仅排在本次检索中的第三位(9%),但它在多数据源结合方面显示出巨大的潜力。
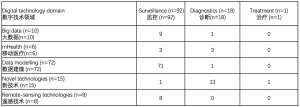
Full table
讨论
本次综述确定了用于应对禽流感的数字技术,其中数据建模占65%,在计算机辅助数学建模参数下,时空建模结合 GPS 和 GIS 功能使用得最多。就禽流感而言,数字技术在通过使用气象数据源跟踪迁徙路线和识别水库来预测潜在爆发热点方面特别有用 [55]。大多数技术用于监测功能,很少用于诊断或治疗目的。尽管禽流感主要影响亚洲国家,但在除中国外的更多北方地区观察到较高的研究产出,中国的研究产出排名第二(见图1)。
在本次综述中数据建模是在最多研究中应用的数字技术,模型能够预测疾病热点的重要变量,几项研究报告指出家禽市场密度和人口密度是所述模型中最重要的预测变量 [28,35,64,80]。时空建模技术还与全球导航卫星系统功能(例如 GIS 和 GPS(24%))相结合,通过绘制鸟类种群的迁徙路线图来确定传播途径和爆发热点[31,71]。数据建模中包含的许多研究都以生态为重点,对与爆发相关的迁移模式进行建模 [28,29,38,40,41,57,64,79,80]。One Health的方法也被纳入模型,通过对物种跨越建模和评估人类感染风险而被注意 [31,57,67,81]。
新技术主要用于诊断目的,包括诊断设备,如具备数十秒内检测目标分子能力的数字微流控设备[99]、RNAi抗病毒载体技术[100]和便携式侧向流装置 [104]。可穿戴传感信号是针对特定情况设定的,可对家禽进行持续监控,并在检测到鸡的异常状态时通过互联网向管理人员发出警报[110]。一项值得注意的研究被归类到治疗领域,该研究通过在鸡身上使用纳米平台的新型疫苗,研究表明与未接种疫苗的鸡相比,接种疫苗的鸡的IgG反应增加[98]。
大数据领域研究主要由社交媒体分析组成,其中包括对 Twitter 等平台的内容挖掘,以及分别参考百度指数和微博的特定国家的搜索引擎和博客 [11,15]。 这些社交媒体平台旨在通过健康交流和增加用户参与来影响行为改变。综述中的一个突出主题是通过基于网络的讨论,使用数据收集,展示参与方法和协作精神。例如,在线数据平台 CaribVnet 和 f-FLUA2H 都分别从普通人群和疾病专家处收集了有关禽流感的信息 [12,14]。 然而,这些平台普遍存在的一个困难是数据质量,这可能因一般人群的成员而异。值得注意的是,大数据也是通过在线学习工具使用和生成的。例如,为专注于禽流感的兽医量身定制的数字学习工具取得了巨大成功,90.2%的参与者认为在线课程有用且方便,97%的参与者希望在他们的职业生涯中应用学到的信息[18]。
遥感和移动医疗领域代表了本次综述中的一部分发现。通过卫星图像的利用,遥感技术在捕捉迁徙模式和潜在热点方面显示出巨大潜力,通过地球卫星观测识别出更多受污染的水体,这些水体充当了禽流感病毒的环境宿主[113,115,116]。此外,遥感技术能够通过迁徙模式记录家禽市场链 [114]。移动医疗主要用于诊断目的,将移动电话设备与成像技术联结起来以形成使用点传感平台 [118],也有将移动设备与荧光技术相结合,用于基于智能手机的荧光诊断系统 [21,22]。
在一些选定的研究[12,79] 中提到目前禽流感防控主要的一个挑战是生物安全措施不佳。例如,加勒比地区和亚洲国家的家禽以自由放养方式为主,在这些国家和地区中,活禽交易市场是很常见的,由于目标是产生销售利润,导致环境卫生的标准清洁通常缺乏严格的监控。此外,考虑与禽流感相关的经济影响也很重要,主要是指活禽/鸟类交易市场和贸易动态。随着对家禽的需求增加,家禽密度和贸易活动也增多了,从而增加了病毒传播的可能性[119]。而嵌在经济影响因素里的是与更多文化习俗相关的潜在人为因素,例如农历新年的庆祝活动。最近的一项研究发现,农历新年期间禽肉消费量从 4.3 倍增加到9.6倍,加剧了需求增加、家禽密度增加以及病毒传播风险增加的循环[6]。
需要注意的是,本综述也存在研究方法的局限性,主要为仅检索了两个数据库(PubMed 和 Web of Science),因此无法涵盖所有相关研究,同时存在发表偏倚。此外,在整个文献收录过程中,因主要关注季节性或流行性感冒,而非禽流感,在整个研究筛选过程中排除了大量文献,这可能是由于在检索关键词中包含了“H2N2”(见附件表S1)。因包含检索关键词导致了部分非标准文献被收录,尤其是综述类文章,从整体上讨论了流感(包括禽流感),尽管大多数结果都集中在大流行性流感上,但也检索出并纳入了有关禽流感和人畜共患病的研究类别里。
结论
数字技术显示出了在改善禽流感检测、控制和预防工作上的潜力。范围界定审查绘制了用于抗击禽流感的现有数字技术,并发现了五个主要的数字领域,包括:移动医疗、大数据、数据建模、遥感和新技术。结果表明数据建模是最常使用的技术,主要用于监测目的。就相关研究产出数量而言,数字技术创新的主要中心包括美国、比利时和中国,这可能是由于资金和高疾病流行率的原因。值得关注的是,尽管通过将计算机辅助模拟与气象和遥感数据集相结合,建模方法已经取得了进步,但仍需要更多创新的方法来发挥其他现有技术的潜力。找到将这些技术结合起来以改进禽流感的治疗和诊断程序的方法仍然至关重要。
Supplementary
表S1
Domain related search terms | Search strategy syntax |
---|---|
Digital technology | “Digital” OR “Technology” OR “Precision medicine” OR “Biosensor” OR “Sensors” OR “Bio-surveillance” OR “Intelligent surveillance” OR “Participatory surveillance” OR “Genomic epidemiology” OR “Genomic sequencing” OR “Pathogen genomics” OR “Big data” OR “Data storage” OR “Data science” OR “Information processing” OR “Blockchain” OR “Social media” OR “Twitter” OR “Facebook” OR “Instagram” OR “Flicker” OR “YouTube” OR “Wikipedia” OR “Telemedicine” OR “Robotics” OR “Machine learning” OR “Modelling” OR “Mathematical |
Avian Influenza | “Influenza in Birds” OR “Influenza, Avian” OR “Fowl Plague” OR “Fowl Plague Virus” OR “Avian Flu” OR “Avian Influenza” OR “Influenza A Virus” OR “Influenza Viruses Type A” OR “Orthomyxovirus Type A” OR “Orthomyxovirus Type A, Avian” OR “Avian Orthomyxovirus Type A” OR “Pestis galli Myxovirus” OR “Myxovirus pestis galli” OR “A (H5N1)” OR “A (H7N9)” OR “A (H9N2)” OR “A (H1N1)” OR “A (H2N2)”OR “Bird Flu” |
Acknowledgments
We would like to acknowledge the Institute of Global Health, Faculty of Medicine at the University of Geneva who supported this work. We would also like to acknowledge and thank Sharada Prasanna Mohanty for his contribution through the production of the authors’ affiliation network.
Funding: None.
Footnote
Provenance and Peer Review: This article was commissioned by the Guest Editor (Mohamed H. Ahmed, Heitham Awadalla and Ahmed O. Almobarak) for the series “The Role of Sudanese Diaspora and NGO in Health System in Sudan” published in Journal of Public Health and Emergency. The article has undergone external peer review.
Conflicts of Interest: All authors have completed the ICMJE uniform disclosure form (available at http://dx.doi.org/10.21037/jphe.2018.06.01). The series “Precision Infectious Disease Epidemiology” was commissioned by the editorial office without any funding or sponsorship. AF serves as an unpaid editorial board member of Journal of Public Health and Emergency from Apr 2018 to Mar 2020 and served as the unpaid Guest Editor of the series. The authors have no other conflicts of interest to declare.
Ethical Statement: The authors are accountable for all aspects of the work in ensuring that questions related to the accuracy or integrity of any part of the work are appropriately investigated and resolved.
Open Access Statement: This is an Open Access article distributed in accordance with the Creative Commons Attribution-NonCommercial-NoDerivs 4.0 International License (CC BY-NC-ND 4.0), which permits the non-commercial replication and distribution of the article with the strict proviso that no changes or edits are made and the original work is properly cited (including links to both the formal publication through the relevant DOI and the license). See: https://creativecommons.org/licenses/by-nc-nd/4.0/.
References
- World Health Organization. Avian and other zoonotic influenza 2017. Available online: http://www.who.int/influenza/human_animal_interface/en/, last accessed 07/09/2017.
- Alexander DJ, Capua I. Avian influenza in poultry. World's Poult Sci J 2008;64:513-26. [Crossref]
- Alexander DJ. An overview of the epidemiology of avian influenza. Vaccine 2007;25:5637-44. [Crossref] [PubMed]
- Barman S, Marinova-Petkova A, Hasan MK, et al. Role of domestic ducks in the emergence of a new genotype of highly pathogenic H5N1 avian influenza A viruses in Bangladesh. Emerg Microbes & Infect 2017;6:e72 [Crossref] [PubMed]
- Chan PK. Outbreak of Avian Influenza A(H5N1) Virus Infection in Hong Kong in 1997. Clin Infect Dis 2002;34:S58-64. [Crossref] [PubMed]
- Yang Y, Halloran ME, Sugimoto JD, et al. Detecting human-to-human transmission of avian influenza A (H5N1). Emerg Infect Dis 2007;13:1348-53. [Crossref] [PubMed]
- Food and Agricultural Organization, World Organization for Animal Health and World Health Organization. The Joint FAO–OIE–WHO Global Early Warning System for health threats and emerging risks at the human–animal–ecosystems interface: A concept paper 2013. Available online: http://www.fao.org/3/a-i3579e.pdf, last accessed 30/05/2018
- World Organisation for Animal Health. Terrestrial Animal Health Code 2017. Available online: http://www.oie.int/standard-setting/terrestrial-code/, last accessed 30/05/2018.
- Colquhoun HL, Levac D, O'Brien KK, et al. Scoping reviews: time for clarity, definitions, methods and reporting. J Clin Epidemiol 2014;67:1291-4. [Crossref] [PubMed]
- Kim P, Sorcar P, Um S, et al. Effects of episodic variations in web-based avian influenza education: influence of fear and humor on perception, comprehension, retention and behavior. Health Educ Res 2009;24:369-80. [Crossref] [PubMed]
- Fung IC, Fu KW, Ying Y, et al. Chinese social media reaction to the MERS-CoV and avian influenza A(H7N9) outbreaks. Infect Dis Poverty 2013;2:31. [Crossref] [PubMed]
- Lefrançois T, Hendrikx P, Erhardt N, et al. Surveillance of avian influenza in the Caribbean through the Caribbean Animal Health Network: surveillance tools and epidemiologic studies. Avian Dis 2010;54:369-73. [Crossref] [PubMed]
- Robertson C, Yee L. Avian Influenza Risk Surveillance in North America with Online Media. PLoS One 2016;11:e0165688 [Crossref] [PubMed]
- Sjaugi MF, Tan S, Abd Raman HS, et al. g-FLUA2H: a web-based application to study the dynamics of animal-to-human mutation transmission for influenza viruses. BMC Med Genomics 2015;8:S5. [Crossref] [PubMed]
- Xie T, Yang Z, Yang S, et al. Correlation between reported human infection with avian influenza A H7N9 virus and cyber user awareness: what can we learn from digital epidemiology? Int J Infect Dis 2014;22:1-3. [Crossref] [PubMed]
- Mao C, Wu XY, Fu XH, et al. An internet-based epidemiological investigation of the outbreak of H7N9 Avian influenza A in China since early 2013. J Med Internet Res 2014;16:e221 [Crossref] [PubMed]
- Willeberg P, Perez A, Thurmond M, et al. Visualization and analysis of the Danish 2006 highly pathogenic avian influenza virus H5N1 wild bird surveillance data by a prototype avian influenza BioPortal. Avian Dis 2010;54:433-9. [Crossref] [PubMed]
- Dalla Pozza M, Valerii L, Graziani M, et al. An Electronic Learning Course on Avian Influenza in Italy (2008). Avian Dis 2010;54:784-6. [Crossref] [PubMed]
- Claes F, Kuznetsov D, Liechti R, et al. The EMPRES-i genetic module: a novel tool linking epidemiological outbreak information and genetic characteristics of influenza viruses. Database (Oxford) 2014;2014:bau008 [Crossref] [PubMed]
- Im H, Park YI, Pathania D, et al. Digital diffraction detection of protein markers for avian influenza. Lab Chip 2016;16:1340-5. [Crossref] [PubMed]
- Lin Y, Heffernan C. Accessible and inexpensive tools for global HPAI surveillance: A mobile-phone based system. Prev Vet Med 2011;98:209-14. [Crossref] [PubMed]
- Yeo SJ, Choi K, Cuc BT, et al. Smartphone-Based Fluorescent Diagnostic System for Highly Pathogenic H5N1 Viruses. Theranostics 2016;6:231-42. [Crossref] [PubMed]
- Yeo SJ, Cuc BT, Sung HW, et al. Evaluation of a smartphone-based rapid fluorescent diagnostic system for H9N2 virus in specific-pathogen-free chickens. Arch Virol 2016;161:2249-56. [Crossref] [PubMed]
- Stephenson LM, Biggs JS, Sheppeard V, et al. An evaluation of the use of short message service during an avian influenza outbreak on a poultry farm in Young. Commun Dis Intell Q Rep 2016;40:E195-201. [PubMed]
- Kim HR, Kwon YK, Jang I, et al. Pathologic Changes in Wild Birds Infected with Highly Pathogenic Avian Influenza A(H5N8) Viruses, South Korea, 2014. Emerg Infect Dis 2015;21:775-80. [Crossref] [PubMed]
- Belkhiria J, Alkhamis MA, Martinez-Lopez B. Application of Species Distribution Modeling for Avian Influenza surveillance in the United States considering the North America Migratory Flyways. Sci Rep 2016;6:33161. [Crossref] [PubMed]
- Bui CM, Gardner L, MacIntyer CR, et al. Influenza A H5N1 and H7N9 in China: A spatial risk analysis. PLoS One 2017;12:e0174980 [Crossref] [PubMed]
- Christensen J, El Allaki F, Vallieres A. Adapting a scenario tree model for freedom from disease as surveillance progresses: The Canadian notifiable avian influenza model. Prev Vet Med 2014;114:132-44. [Crossref] [PubMed]
- Dhingra MS, Artois J, Robinson TP, et al. Global mapping of highly pathogenic avian influenza H5N1 and H5Nx clade 2.3.4.4 viruses with spatial cross-validation. Elife 2016;5. [PubMed]
- Gilbert M, Golding N, Zhou H, et al. Predicting the risk of avian influenza A H7N9 infection in live-poultry markets across Asia. Nat Commun 2014;5:4116. [Crossref] [PubMed]
- Li X, Liu X, Xu L, et al. Spatial transmission of avian influenza (type H5) in birds. Integr Zool 2009;4:418-25. [Crossref] [PubMed]
- Shi B, Xia S, Yang GJ, et al. Inferring the potential risks of H7N9 infection by spatiotemporally characterizing bird migration and poultry distribution in eastern China. Infect Dis Poverty 2013;2:8. [Crossref] [PubMed]
- Martcheva M. Avian Flu: Modeling and implications for control. J Bio Sys 2014;22:151-75. [Crossref]
- Alkhamis M, Perez A, Batey N, et al. Modeling the Association of Space, Time, and Host Species with Variation of the HA, NA, and NS Genes of H5N1 Highly Pathogenic Avian Influenza Viruses Isolated from Birds in Romania in 2005-2007. Avian Dis 2013;57:612-21. [Crossref] [PubMed]
- Artois J, Lai S, Feng L, et al. H7N9 and H5N1 avian influenza suitability models for China: accounting for new poultry and live-poultry markets distribution data. Stoch Environ Res Risk Assess 2017;31:393-402. [Crossref] [PubMed]
- Cador C, Rose N, Willem L, et al. Maternally Derived Immunity Extends Swine Influenza A Virus Persistence within Farrow-to-Finish Pig Farms: Insights from a Stochastic Event-Driven Metapopulation Model. PLoS One 2016;11:e0163672 [Crossref] [PubMed]
- Cappelle J, Girard O, Fofana B, et al. Ecological modeling of the spatial distribution of wild waterbirds to identify the main areas where avian influenza viruses are circulating in the Inner Niger Delta, Mali. Ecohealth 2010;7:283-93. [Crossref] [PubMed]
- Chong NS, Tchuenche JM, Smith RJ. A mathematical model of avian influenza with half-saturated incidence. Theory Biosci 2014;133:23-38. [Crossref] [PubMed]
- Farnsworth ML, Fitchett S, Hidayat MM, et al. Metapopulation dynamics and determinants of H5N1 highly pathogenic avian influenza outbreaks in Indonesian poultry. Prev Vet Med 2011;102:206-17. [Crossref] [PubMed]
- Hernandez-Jover. Evaluating the risk of avian influenza introduction and spread among poultry exhibition flocks in Australia. Prev Vet Med 2015;118:128-41. [Crossref] [PubMed]
- Hill EM, House T, Dhingra MS, et al. Modelling H5N1 in Bangladesh across spatial scales: Model complexity and zoonotic transmission risk. Epidemics 2017;20:37-55. [Crossref] [PubMed]
- Lu J, Liu W, Xia R, et al. Effects of closing and reopening live poultry markets on the epidemic of human infection with avian influenza A virus. J Biomed Res 2016;30:112-9. [PubMed]
- Moriguchi S, Onuma M, Goka K. Potential risk map for avian influenza A virus invading Japan. Diversity and Distributions 2013;19:78-85. [Crossref]
- Nickbakhsh S, Hall M, Dorigatti I, et al. Modelling the impact of co-circulating low pathogenic avian influenza viruses on epidemics of highly pathogenic avian influenza in poultry. Epidemics 2016;17:27-34. [Crossref] [PubMed]
- Paul M, Tarvonparnich S, Abrial D, et al. Anthropogenic factors and the risk of highly pathogenic avian influenza H5N1: prospects from a spatial-based model. Vet Res 2010;41:28. [Crossref] [PubMed]
- Pelletier STK, Rorres S, Macko PC, et al. Models of highly pathogenic avian influenza epidemics in commercial poultry flocks in Nigeria and Ghana. Trop Anim Health Prod 2012;44:1681-7. [Crossref] [PubMed]
- Reynolds JJH, Torremorell M, Craft ME. Mathematical Modeling of Influenza A Virus Dynamics within Swine Farms and the Effects of Vaccination. PLoS One 2014;9:e106177 [Crossref] [PubMed]
- Stevens KB, Gilbert M, Pfeiffer DU. Modeling habitat suitability for occurrence of highly pathogenic avian influenza virus H5N1 in domestic poultry in Asia: a spatial multicriteria decision analysis approach. Spat Spatiotemporal Epidemiol 2013;4:1-14. [Crossref] [PubMed]
- Takekawa JY, Hill NJ, Schultz AK, et al. Rapid diagnosis of avian influenza virus in wild birds: use of a portable rRT-PCR and freeze-dried reagents in the field. J Vis Ex 2011;(54).
- Van Boeckel TP, Thanapngtharm W, Robinson T, et al. Improving risk models for avian influenza: the role of intensive poultry farming and flooded land during the 2004 Thailand epidemic. PLoS One 2012;7:e49528 [Crossref] [PubMed]
- Vittecoq M, Gaudin H, Oudart T, et al. Modeling the spread of avian influenza viruses in aquatic reservoirs: A novel hydrodynamic approach applied to the Rhone delta (southern France). Sci Total Environ 2017;595:787-800. [Crossref] [PubMed]
- Wiratsudakul A, Paul MC, Bicout DJ, et al. Modeling the dynamics of backyard chicken flows in traditional trade networks in Thailand: implications for surveillance and control of avian influenza. Trop Anim Health Prod 2014;46:845-53. [Crossref] [PubMed]
- Goutard FL, Paul M, Tavornpanich S, et al. Optimizing early detection of avian influenza H5N1 in backyard and free-range poultry production systems in Thailand. Prev Vet Med 2012;105:223-34. [Crossref] [PubMed]
- Yu H, Wu JT, Cowling BJ, et al. Effect of closure of live poultry markets on poultry-to-person transmission of avian influenza A H7N9 virus: an ecological study. Lancet 2014;383:541-8. [Crossref] [PubMed]
- Biswas PK, Islam Z, Debnth NC, et al. Modeling and roles of meteorological factors in outbreaks of highly pathogenic avian influenza H5N1. PLoS One 2014;9:e98471 [Crossref] [PubMed]
- Boni MF, Manh BH, Thai Q, et al. Modelling the progression of pandemic influenza A (H1N1) in Vietnam and the opportunities for reassortment with other influenza viruses. BMC Med 2009;7:43. [Crossref] [PubMed]
- Dhingra MS, Dissanayake R, Negi AB, et al. Spatio-temporal epidemiology of highly pathogenic avian influenza (subtype H5N1) in poultry in eastern India. Spat Spatiotemporal Epidemiol 2014;11:45-57. [Crossref] [PubMed]
- Hénaux V, Samuel MD, Bunck CM. Model-Based Evaluation of Highly and Low Pathogenic Avian Influenza Dynamics in Wild Birds. PLoS One 2010;5:e10997 [Crossref] [PubMed]
- Loth L, Gilbert M, Osmani MG, et al. Risk factors and clusters of Highly Pathogenic Avian Influenza H5N1 outbreaks in Bangladesh. Prev Vet Med 2010;96:104-13. [Crossref] [PubMed]
- Nishiguchi A, Kobyashi S, Ouchi Y, et al. Spatial Analysis of Low Pathogenic H5N2 Avian Influenza Outbreaks in Japan in 2005. J Vet Med Sci 2009;71:979-82. [Crossref] [PubMed]
- Prosser DJ, Hungerford LL. Spatial Modeling of Wild Bird Risk Factors for Highly Pathogenic A(H5N1) Avian Influenza Virus Transmission. Avian Dis 2016;60:329-36. [Crossref] [PubMed]
- Rao DM, Chernyakhovsky A, Rao V. Modeling and analysis of global epidemiology of avian influenza. Environmental Modelling & Software 2009;24:24-134. [Crossref]
- Takekawa JY, Prosser DJ, Collins BM, et al. Movements of wild ruddy shelducks in the Central Asian Flyway and their spatial relationship to outbreaks of highly pathogenic avian influenza H5N1. Viruses 2013;5:2129-52. [Crossref] [PubMed]
- Ekong PS, Ducheyne E, Carpenter TE, et al. Spatio-temporal epidemiology of highly pathogenic avian influenza (H5N1) outbreaks in Nigeria, 2006-2008. Prev Vet Med 2012;103:170-7. [Crossref] [PubMed]
- Farnsworth ML, Ward MP. Identifying spatio-temporal patterns of transboundary disease spread: examples using avian influenza H5N1 outbreaks. Vet Res 2009;40:20. [Crossref] [PubMed]
- Hagenaars TJ, Fischer EA, Jansen JM, et al. Modelling the Innate Immune Response against Avian Influenza Virus in Chicken. PLoS One 2016;11:e0157816 [Crossref] [PubMed]
- Herrick KA, Huettmann F, Lindgren MA. A global model of avian influenza prediction in wild birds: the importance of northern regions. Vet Res 2013;44:42. [Crossref] [PubMed]
- Kitajima M, Huang Y, Watanabe T, et al. Dose-response time modelling for highly pathogenic avian influenza A (H5N1) virus infection. Lett Appl Microbiol 2011;53:438-44. [Crossref] [PubMed]
- Liang L, Xu B, Chen Y, et al. Combining spatial-temporal and phylogenetic analysis approaches for improved understanding on global H5N1 transmission. PLoS One 2010;5:e13575 [Crossref] [PubMed]
- Marquetoux N, Paul M, Wognarkpet S, et al. Estimating spatial and temporal variations of the reproduction number for highly pathogenic avian influenza H5N1 epidemic in Thailand. Prev Vet Med 2012;106:143-51. [Crossref] [PubMed]
- Prosser DJ, Hungerford LL, Erwin RM, et al. Mapping avian influenza transmission risk at the interface of domestic poultry and wild birds. Front Public Health 2013;1:28. [Crossref] [PubMed]
- Rorres C, Pelletier ST, Bruhn MC, et al. Ongoing estimation of the epidemic parameters of a stochastic, spatial, discrete-time model for a 1983-84 avian influenza epidemic. Avian Dis 2011;55:35-42. [Crossref] [PubMed]
- Van Boeckel TP, Thanapngtharm W, Robinson T, et al. Predicting the distribution of intensive poultry farming in Thailand. Agric Ecosyst Environ 2012;149:144-53. [Crossref] [PubMed]
- Zhang Y, Shen Z, Ma C, et al. Cluster of human infections with avian influenza A (H7N9) cases: a temporal and spatial analysis. Int J Environ Res Public Health 2015;12:816-28. [Crossref] [PubMed]
- Si Y, Skidmore AK, Wang T, et al. Spatio-temporal dynamics of global H5N1 outbreaks match bird migration patterns. Geospat Health 2009;4:65-78. [Crossref] [PubMed]
- Minh PQ, Morris RS, Schauer B, et al. Spatio-temporal epidemiology of highly pathogenic avian influenza outbreaks in the two deltas of Vietnam during 2003-2007. Prev Vet Med 2009;89:16-24. [Crossref] [PubMed]
- Si Y, Skidmore AK, Wang T, et al. Spatio-temporal dynamics of global H5N1 outbreaks match bird migration patterns. Geospat Health 2009;4:65-78. [Crossref] [PubMed]
- Bodbyl-Roels S, Peterson AT, Xiao X. Comparative analysis of remotely-sensed data products via ecological niche modeling of avian influenza case occurrences in Middle Eastern poultry. Int J Health Geogr 2011;10:21. [Crossref] [PubMed]
- Dorjee S, Revie CW, Poljak Z, et al. One-Health Simulation Modelling: Assessment of Control Strategies Against the Spread of Influenza between Swine and Human Populations Using NAADSM. Transbound Emerg Dis 2016;63:e229-e244. [Crossref] [PubMed]
- Fang LQ, Li XL, Liu K, et al. Mapping spread and risk of avian influenza A (H7N9) in China. Sci Rep 2013;3:2722. [Crossref] [PubMed]
- Fasina FO, Njage PM, Ali AM, et al. Development of Disease-specific, Context-specific Surveillance Models: Avian Influenza (H5N1)-Related Risks and Behaviours in African Countries. Zoonoses and Public Health 2016;63:20-33. [Crossref] [PubMed]
- Hill AA, Dewè T, Kosmider R, et al. Modelling the species jump: towards assessing the risk of human infection from novel avian influenzas. R Soc Open Sci 2015;2:150173 [Crossref] [PubMed]
- Jewell CP, Kypraios T, Christley RM, et al. A novel approach to real-time risk prediction for emerging infectious diseases: a case study in Avian Influenza H5N1. Prev Vet Med 2009;91:19-28. [Crossref] [PubMed]
- Li XL, Yang Y, Sun Y, et al. Risk Distribution of Human Infections with Avian Influenza H7N9 and H5N1 virus in China. Sci Rep 2015;5:18610. [Crossref] [PubMed]
- Liu S, Ruan S, Zhang X. Nonlinear dynamics of avian influenza epidemic models. Mathematical Biosciences 2017;283:118-35. [Crossref] [PubMed]
- Martin V, Pfeiffer D, Zhou X, et al. Spatial distribution and risk factors of highly pathogenic avian influenza (HPAI) H5N1 in China. PLoS Pathog 2011;7:e1001308 [Crossref] [PubMed]
- Ojimelukwe AE, Prakarnkamanant A, Rushton J. Estimating the sensitivity of passive surveillance for HPAI H5N1 in Bayelsa state, Nigeria. Prev Vet Med 2016;129:58-66. [Crossref] [PubMed]
- Paul MC, Gourtard FL, Rolleau F, et al. Quantitative assessment of a spatial multicriteria model for highly pathogenic avian influenza H5N1 in Thailand, and application in Cambodia. Sci Rep 2016;6:31096. [Crossref] [PubMed]
- Prosser DJ, Palm EC, Takekawa JY, et al. Movement analysis of free-grazing domestic ducks in Poyang Lake, China: a disease connection. Int J Geogr Inf Sci 2016;30:869-80. [Crossref] [PubMed]
- Ssematimba A, Hagenaars TJ, de Jong MC. Modelling the wind-borne spread of highly pathogenic avian influenza virus between farms. PLoS One 2012;7:e31114 [Crossref] [PubMed]
- Tian H, Dong L, Zhou S, et al. Spatial, temporal and genetic dynamics of highly pathogenic avian influenza A (H5N1) virus in China. BMC Infect Dis 2015;15:54. [Crossref] [PubMed]
- Van Boeckel TP, Prosser D, Fanceschini G, et al. Modelling the distribution of domestic ducks in Monsoon Asia. Agric Ecosyst Environ 2011;141:373-80. [Crossref] [PubMed]
- Zhang Z, Chen D, Ward MP, et al. Transmissibility of the highly pathogenic avian influenza virus, subtype H5N1 in domestic poultry: a spatio-temporal estimation at the global scale. Geospat Health 2012;7:135-43. [Crossref] [PubMed]
- Bentley RA, Ormerod P. A rapid method for assessing social versus independent interest in health issues: a case study of 'bird flu' and 'swine flu'. Soc Sci Med 2010;71:482-5. [Crossref] [PubMed]
- Loth L, Gilbertb M, Mozaffar MG, et al. Risk factors and clusters of Highly Pathogenic Avian Influenza H5N1 outbreaks in Bangladesh. Prev Vet Med 2010;96:104-13. [Crossref] [PubMed]
- Ward MP, Maftei D, Apostu C, et al. Environmental and anthropogenic risk factors for highly pathogenic avian influenza subtype H5N1 outbreaks in Romania, 2005--2006. Vet Res Commun 2008;32:627-34. [Crossref] [PubMed]
- Kim KI, Lin Z, Zhang L. Avian-human influenza epidemic model with diffusion. Nonlinesuiar Analysis: Real World Applications 2010;11:313-22. [Crossref]
- Babapoor S, Neef T, Mittleholzer C, et al. A Novel Vaccine Using Nanoparticle Platform to Present Immunogenic M2e against Avian Influenza Infection. Influenza Res Treat 2011;126794 [PubMed]
- Choi K, Kim JY, Ahn JH, et al. Integration of field effect transistor-based biosensors with a digital microfluidic device for a lab-on-a-chip application. Lab Chip 2012;12:1533-9. [Crossref] [PubMed]
- Huang J, Xie Z, Xie Z, et al. Silver nanoparticles coated graphene electrochemical sensor for the ultrasensitive analysis of avian influenza virus H7. Anal Chim Acta 2016;913:121-7. [Crossref] [PubMed]
- Karash S, Wang R, Kelso L, et al. Rapid detection of avian influenza virus H5N1 in chicken tracheal samples using an impedance aptasensor with gold nanoparticles for signal amplification. J Virol Methods 2016;236:147-56. [Crossref] [PubMed]
- Linke LM, Wilusz J, Pabilonia KL, et al. Inhibiting avian influenza virus shedding using a novel RNAi antiviral vector technology: proof of concept in an avian cell model. Amb Express 2016;6:16. [Crossref] [PubMed]
- Nagy A, Cerníková L, Křivda V, et al. Digital genotyping of avian influenza viruses of H7 subtype detected in central Europe in 2007-2011. Virus Res 2012;165:126-33. [Crossref] [PubMed]
- Soliman M, Selimi M, Coward VJ, et al. Evaluation of two commercial lateral flow devices (LFDs) used for flockside testing of H5N1 highly-pathogenic avian influenza infections in backyard gallinaceous poultry in Egypt. J Mol Genet Med 2010;4:247-51. [Crossref] [PubMed]
- Takekawa JY, Hill, NJ, Schultz AK, et al. Rapid diagnosis of avian influenza virus in wild birds: use of a portable rRT-PCR and freeze-dried reagents in the field. J Vis Exp 2011;(54).
- Vidic J, Manzano M, Chang CM, et al. Advanced biosensors for detection of pathogens related to livestock and poultry. Vet Res 2017;48:11. [Crossref] [PubMed]
- Yan Y, Jia XY, Wang HH, et al. Dynamic quantification of avian influenza H7N9(A) virus in a human infection during clinical treatment using droplet digital PCR. J Virol Methods 2016;234:22-7. [Crossref] [PubMed]
- Ahn JH, Im M, Park TJ, et al. Label-Free and Real-Time Detection of Avian Influenza Using Nanowire Field Effect Transistors. J Biomed Nanotechnol 2015;11:1640-3. [Crossref] [PubMed]
- Lum J, Wang R, Hagris B, et al. An Impedance Aptasensor with Microfluidic Chips for Specific Detection of H5N1 Avian Influenza Virus. Sensors (Basel) 2015;15:18565-78. [Crossref] [PubMed]
- Okada H, Nogami H, Kobyashi T, et al. Avian Influenza Surveillance System with Wearable Wireless Sensor Node Using Pb(Zr, Ti)O-3 Microcantilever. Sensors and Materials 2013;25:619-26.
- Zou X, Huang H, Gao Y, et al. Detection of avian influenza virus based on magnetic silica nanoparticles resonance light scattering system. Analyst 2012;137:648-53. [Crossref] [PubMed]
- Abd El Wahed A, Weidmann M, Hufert FT. Diagnostics-in-a-Suitcase: Development of a portable and rapid assay for the detection of the emerging avian influenza A (H7N9) virus. J Clin Virol 2015;69:16-21. [Crossref] [PubMed]
- Bridge ES, Kelly FS, Xiao X, et al. Bird Migration and Avian Influenza: A Comparison of Hydrogen Stabl Isotopes and Satellite Tracking Methods. Ecol Indic 2014;45:266-73. [Crossref] [PubMed]
- Choi CY, Takekawa J, Xiong Y, et al. Tracking domestic ducks: A novel approach for documenting poultry market chains in the context of avian influenza transmission. J Int Agri 2016;15:1584-94. [Crossref]
- Gaidet N, Cappelle J, Takekawa JY, et al. Potential spread of highly pathogenic avian influenza H5N1 by wildfowl: dispersal ranges and rates determined from large-scale satellite telemetry. J App Ecolo 2010;47:1147-57. [Crossref]
- Gilbert M, Newman S, Takekawa JY, et al. Flying over an infected landscape: distribution of highly pathogenic avian influenza H5N1 risk in South Asia and satellite tracking of wild waterfowl. Ecohealth 2010;7:448-58. [Crossref] [PubMed]
- Guerrini L, Paul MC, Leger L, et al. Landscape attributes driving avian influenza virus circulation in the Lake Alaotra region of Madagascar. Geospat Health 2014;8:445-53. [Crossref] [PubMed]
- Tran A, Goutard F, Chamaillé L, et al. Remote sensing and avian influenza: A review of image processing methods for extracting key variables affecting avian influenza virus survival in water from Earth Observation satellites. International Journal of Applied Earth Observation and Geoinformation 2010;12:1-8. [Crossref]
- Delabouglise A, Choisy M, Phan TD, et al. Economic factors influencing zoonotic disease dynamics: demand for poultry meat and seasonal transmission of avian influenza in Vietnam. Sci Rep 2017;7:5905. [Crossref] [PubMed]
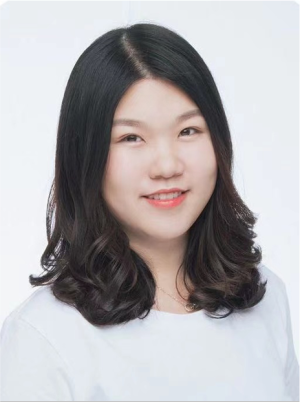
钟韵
毕业于中央民族大学(985,211)生物科学,硕士2012年毕业于香港大学李嘉诚医学院,公共卫生硕士(MPH),研究方向为健康经济政策与流行病学。毕业后在香港从事医疗市场和医疗投资工作。2020年参与哈佛大学公共卫生学院Global Health Intensive Program项目,与全球公共卫生领域专家与青年学者共同参与新冠肺炎疫情全球控制和全球健康应对政策讨论。(更新时间:2021/9/10)
(本译文仅供学术交流,实际内容请以英文原文为准。)
Cite this article as: Bempong NE, Ruiz De Castañeda R, Dietrich D, Bolon I, Flahault A. Taking flight with Precision Global Health: a scoping review on avian influenza. J Public Health Emerg 2018;2:21.