Assessment of state socioeconomic and demographic disparities on coronavirus-2019 mortality during the exponential increase period in the US
Introduction
The coronavirus disease-19 (COVID-19) pandemic began in December 2019 in Wuhan, China and rapidly spread worldwide resulting in over 3 million confirmed cases and 300,000 deaths (1,2). The World Health Organization (WHO) declared a global pandemic on March 11, 2020. In the United States, the first case was reported on January 20, 2020. The U.S. has over a million confirmed cases and 80,000 deaths, with most of them in the New York City metropolitan area (>300,000 cases and >25,000 deaths) by May 31, 2020 (3,4). The most common symptoms are fever, cough and dyspnea (5,6). About 20% of confirmed cases developed acute respiratory distress syndrome (ARDS), particularly older adults with serious underlying health conditions. Approximately 3% of them exhibited pulmonary edema and lung failure, and have liver, heart, and kidney damage (1,6). Lung computed tomography (CT) scans of COVID-19 patients were described as bilateral, subpleural, ground-glass opacities with air bronchograms, ill-defined margins, and a slight predominance in the right lower lobe even in asymptomatic patients, with rapidly developing lesions about two weeks after onset (7,8). COVID-19 patients also presented with a cytokine storm with elevated serum levels of several cytokines such as IL-1β, IL-17, GM-CSF and TNF-α, causing tissue damage and pulmonary edema (6)
Preliminary assessment of deaths in hospitals and/or regions showed that hospitalized patients were predominantly older males with a mortality rate of higher than 25% in China, Italy and New York City (9-11). However, there are significant differences in population demographics and socioeconomic characteristics among the three most impacted regions by May 31, 2020. There are preliminary indications that minorities have been disproportionally affected by COVID-19 (12,13). In fact, more than 50% of deaths in Wisconsin and Chicago, Illinois were African-Americans, despite that African-Americans account for less than 30% of the population (14). Assessing racial and socioeconomic disparities of COVID-19 in the midst of pandemic are challenging because of poor and irregular tracking of race and socioeconomic characteristics.
The objective of the study was to evaluate the effect of population demographics and socioeconomic characteristics on COVID-19 death rate during the exponential increase period. Understanding the dynamics of the exponential increase period is of importance to control the spread of the disease and ensure adequate healthcare capacity and quality is maintained. For this analysis, statewide COVID-19 cases and deaths as reported by state, county and city health departments were used. Although COVID-19 cases and deaths may have varied substantially within a state; state-wide social distancing policies and measures initially implemented such as the closure of educational facilities and gathering restrictions and trends were representative of state outcomes. It also prevented misclassification of the spatial distribution of cases and deaths between the county of residency and county of the hospital in which the case and/or death was recorded. It is noteworthy that the implementation of policies such as the closure of non-essential business may have not been consistent across the US and states.
Daily COVID-19 cases and deaths data were fitted using the Boltzmann sigmoidal function. The curve describes the trend of cases and deaths over time. Boltzmann or logistic sigmoidal models have been developed for non-linear fitting of the natural systems data (15-17). The model has been recently used to predict COVID-19 cases and deaths in China and Brazil (18,19). The advantage of non-linear fitting is that the output (COVID-19 cases or deaths) is not a constant but a variable that changes over time having a maximum in the exponential increase period (i.e., the time interval of rapid COVID-19 cases and deaths increase during the January 22–May 31, 2020 period) and then a plateau to a containment level upon effective implementation of social distancing measures, thus generating a sigmoidal curve. We present the following article in accordance with the MDAR checklist (available at http://dx.doi.org/10.21037/jphe-20-96).
Methods
Health data
The study was conducted in accordance with the Declaration of Helsinki (as revised in 2013).
Novel coronavirus cases and deaths from January 22, 2020 to May 31, 2020 were obtained from the Johns Hopkins University Center for Systems Science and Engineering Data Repository (20). Databases were obtained every three days and evaluated for consistency over time. Daily COVID-19 cases of deaths higher than (e-1) (i.e., ~1.7) times the previous day COVID-19 cases or deaths were flagged and assessed. They were attributed to the introduction of new testing technologies at the beginning of the pandemic in the US that increased the number of positive tests and cumulative reports of deaths at home or non-clinical facilities (e.g., nursing homes), respectively. The crude incidence and mortality rates per 100,000 people were calculated for COVID-19 cases and deaths, respectively.
Boltzmann sigmoidal fitting
The Boltzmann sigmoidal function was used to describe the cumulative counts of COVID-19 cases and deaths for each state as a function of time. Curve fitting of the Boltzmann function {Eq. [1]} was done using the Levenberg-Marquardt algorithm in OriginPro (Ver. 9.0) (21), where Yi,k was COVID-19 cases or deaths on i-day in k-th state.
The lowest value (Y0,k) was set to 0.0. The outcomes of the fitting curve were the maximum number of simulated cases or deaths (Ymax,k), ck was the inflection point (where 50% of cases or deaths were reported) and αk is the coefficient that describes the duration of the exponential increase period of COVID-19 cases and deaths for k-th state. The larger the value of αk, the more shallow the exponential increase period indicating a longer period of COVID-19 cases and deaths. The rate of the exponential increase period (Sk) (events/day) per 1,000,000 people was computed using Eq. [2] with large values denoting a rapid spread of COVID-19 infections or mortality, where Popk is the population of k-th state.
The reliability of the Boltzmann curve fit was assessed by the intra-class correlation coefficient between actual and observed cases (>0.75) and the percent relative difference of reported and computed Ymax,k values (>5%).
Population socioeconomic and demographic estimates
The characteristics of population in each state for 2019 were obtained from U.S. Census American Community Survey. They included (I) the percent of people living in urban agglomerations in each state; (II) age (above 45 years old); (III) sex (male); (IV) race (non-white); (V) ethnicity (Hispanics); (VI) percent of people below the poverty level and (VII) uninsured (22). The percent of people older than 45 years was selected to account for the increased COVID-19 susceptibility (both severe cases and/or mortality) of older population. The percent of people living in urban agglomerations was selected as a metric of the density of people living in dense quarters to better describe the population density in large by size states with most of people living a limited number of urban areas (e.g., New York, Texas).
Statistical analysis
A tiered ecological approach was used to assess the relationship between COVID-19 cases and mortality to population characteristics. First, the spatial distribution of inflection point, duration and rate of the exponential increase period (ck, ak and Sk, respectively) of COVID-19 cases and deaths were plotted using QGIS (Ver. 3.14). Secondly, we computed the mean (± SEM) of duration and rate of exponential increase period of COVID-19 cases for states grouped into quintiles by their population characteristics. Tests were conducted using Bonferroni adjusted alpha levels of 0.0125 per test (0.05/4). Finally, ordinary least regression analysis was used to model the relationships between deaths rate, cases rate and population characteristics (as continuous percentages). Significance of the regression coefficient was determined by rejecting the null hypothesis at the 0.05 level. When a regression coefficient did not meet these significance criteria, it was kept in the analysis as an indicator of the tendency. The variance infiltration factor (VIF) was applied to detect multicollinearity for the individual regression coefficients (if VIF >10.0). The intercept represents COVID-19 deaths that were not a result of the infection. We kept it in the analysis as an indicator of regression accuracy with values below zero being indicative of the causal relationship between infections and deaths. This was further supported by the unchanged regression coefficient of cases rate for models with and without intercept. Regression analysis was done using IBM SPSS software (Ver. 25.0). Spatial autocorrelation of the regression residual was assessed using GeoDa software; however, no differences were observed (Moran’s I=−0.003, P<0.001) (23).
Results
COVID-19 cases and deaths
A total of approximately 1.8 million and 100,000 COVID-19 cases and deaths were reported by May 31, 2020, respectively. The mean crude rates for confirmed COVID-19 cases and deaths were 244.3±29.8 and 12.7±2.3 deaths per 100,000 with an average of 5% mortality rate. At the beginning of the pandemic, COVID-19 diagnosis was limited due to the lack of sufficient and robust testing. As new tests were developed, testing continued to be prioritized for those who had severe respiratory distress symptoms and healthcare personnel. Recent estimates based on antibody testing showed that the infection rate may have been three times higher than previously determined (24,25). Similarly, COVID-19 deaths may be underestimated because only deaths in hospitals of confirmed COVID-19 cases were reported on a daily basis. Deaths in nursing and hospice facilities were irregularly reported. Misclassification of death cause may be possible particularly for elderly with underlying medical conditions. Furthermore, deaths in homes were not included.
From these trends, the Boltzmann sigmoidal fitting was drawn for daily cumulative counts. Figure 1A,B presents the cumulative COVID-19 cases and deaths for the four most populous states in the US (California, Texas, Florida and New York). For all states, the Boltzmann function fitted quite well COVID-19 cases (mean ± SEM of coefficient of determination; R2>0.98±0.01) and deaths (R2>0.99±0.01). The cumulative simulated COVID-19 cases and deaths (1,853,811 and 104,753 deaths) were slightly higher (percent difference of 5.6 for cases and 4.8 for deaths) than those reported by May 31, 2020 (Table 1).
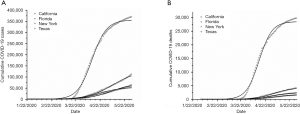
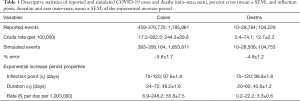
Full table
Spatial variation of COVID-19 exponential increase period characteristics
The duration of the exponential increase period varied from 24 to 72 with an average of 49.2 days for COVID-19 cases and from 20 to 60 with an average of 43.6 for COVID-19 deaths indicating similar temporal trends for cases and deaths (Table 1). Shorter duration may be associated with unique local circumstances such as the effective control of community spread, through implementation of social distancing policies (for Tri-state) and slow spread due to low population density (Idaho and Montana) (Figure 2A,B). This was also supported by the variability of the exponential increase period duration for COVID-19 deaths. For example, for the Tri-state region, the combination of high COVID-19 cases and short exponential increase periods suggested the potential for overcrowding of health care facilities. On the contrary, low COVID-19 cases for longer exponential increase periods suggested a slower spread of the infection and the possibility for prolonged presence of COVID-19. Taking into account the inflection point and exponential increase period for the most populous states (California, Texas and to a lesser extend for Florida), there is a potential for more cases and deaths in these states in the near future that can be further augmented by relaxation of social distancing measures (Figure 2A,B,C,D). This appears to be the case with the delayed growth of COVID-19 cases across the US in June-August 2020.
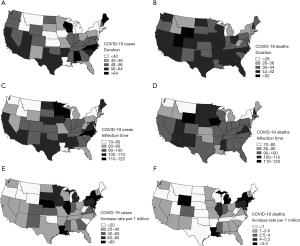
The inflection points for both cases and deaths were comparable (97.6±1.8 days and 98.8±1.8 days) and reached within 80 days in the Tri-state (New York, New Jersey and Connecticut), Northwest (Washington and neighboring Idaho and Montana) and Louisiana (Figure 1A,B). COVID-19 cases reported in California were associated with the relocation of cruise ship passengers and Wuhan residents; however, community spread was limited. Higher inflection values were computed for Great Plains (Minnesota, Wisconsin, North and South Dakota, Nebraska and Iowa) and Southern states (Mississippi, Texas, Arizona, New Mexico) due to delayed introduction of COVID-19 as compared to other states (Figure 2C,D).
The exponential increase period rate for COVID-19 cases and deaths were 55.8±7.5 and 3.3±0.6 per day per 1,000,000 residents with the highest values measured in the Tri-state region and neighboring states and Louisiana. The lowest death rates were calculated for Great Plains, along the Tennessee Valley and Northwest states (except for Washington) (more than 2 standard deviation of the average rate for the remaining states) (Figure 2E,F). Access and utilization of emergency health care services may also account for the low deaths rates particularly in geographically large and rural states.
Despite the expansion of testing, it is likely that testing capacity in rural areas was limited and did not meet needs in urban areas, too (26). Emergency care may have been utilized more often in urban areas due to close proximity as compared to rural areas (27). It has been previously determined that residents in rural communities live an average of 17 km from the nearest hospital with considerable variability, as compared with 7.0 km for people in urban areas. It takes an average of 34 minutes to get to the nearest acute care facility for about a quarter of residents in rural communities in the Great Plains region (28). Utilization of emergency health care may also have been suppressed by lack of health care insurance. Reduced mortality rates were observed in countries that implemented strict social distancing policies and/or developed widespread testing such as Germany, China and Greece, with publicly-run health care facilities (29).
Effect of socioeconomic and demographic profiles on COVID-19 cases
Table 2 shows the average ± SEM of COVID-19 cases exponential increase period duration and rate for states ranked by the percentage of population living in urban areas, without health insurance, below poverty level, males, older than 45 years, minorities, and of Hispanic origin. The rate of the exponential increase period was significantly higher for the most densely populated urban areas (Q4) as compared to areas with the first three quintiles (P<0.001). The pairwise comparison of the exponential increase period for areas within Q1, Q2 and Q3 by urban population density was non-significant. The rate of the exponential increase period was significantly lower for states with the higher percentage of males (Q3 and Q4) as compared to the states with the lowest male population (Q1) (P=0.010). There was no difference on both the duration and mean rate for states grouped based on population older than 45 years, uninsured, non-Whites, Hispanics and living below poverty level.
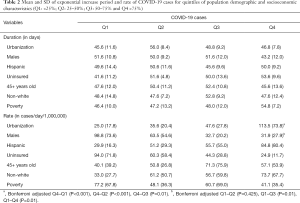
Full table
Effect of socioeconomic and demographic profiles on COVID-19 deaths
The relationship between COVID-19 deaths and cases rates for the exponential increase period and the contribution of population characteristics was assessed for an IQR increase of the dependent variable (Table 3) (R2=0.86). A positive relationship was observed for all variables. The stronger association was observed for the disease rate as expected; an increase of up to 340% for an increase of 43.7 cases per 1,000,000 residences (P<0.001). An increase of more than 50% on the exponential increase period death rate was computed for an IQR range change in the percentage of older than 45 years and minorities, followed by males (a 30% increase). This indicated that the socioeconomic status and profile of affected communities had an immediate impact on COVID-19 mortality, albeit the threshold of statistical significance was not reached. This may be associated with long-term disparities in healthcare access for minorities, particularly those living in large urban areas.
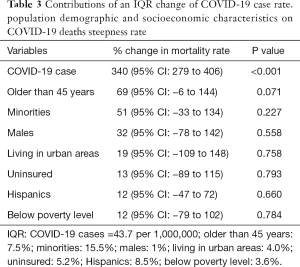
Full table
This study had some limitations. Daily COVID-19 cases and deaths were reported by state, county of city health departments based on data provided to them by health care and related facilities. Although government agencies operate and comply with the same set of federal regulations, it was possible that raw daily counts may have been irregularly reported. However, given that original data were continuously updated and screened, we expect that the potential for systematic error on COVID-19 reports to be small. It is also possible that deaths may have been misclassified, particularly in regions with many daily deaths; however, there is no evidence of substantial under or over-reporting COVID-19 deaths in the literature. The spatial scale of the analysis may also be challenging, given the vast differences in the distribution of COVID-19 cases, deaths and population characteristics within states. Analysis at a finer scale would have been incomplete for the study period, due to relatively low COVID-19 cases and deaths in sparsely populated rural counties (because of the relatively widespread of statewide policies on school closures and gathering restrictions). Given that minorities disproportionally reside in heavily populated urban areas that experienced the highest COVID-19 cases and deaths rates, we anticipate that the use of state-level population characteristics, that includes rural areas with predominantly white residents, may underestimate the effect of race and ethnicity on COVID-19 mortality. Other individual and community-level parameters may also affect COVID-19 spread and mortality. These may include pre-existing medical conditions, smoking, housing, living conditions, time spent indoors or outdoors, environmental factors (temperature, humidity, solar radiation, air quality) affecting the survival of SARS-CoV-2 virus, personal hygiene and activities, and businesses practices. Understanding their effect on COVID-19 spread and mortality require a combination of field, laboratory, biomedical and health-related studies.
Conclusions
In the first wave of COVID-19 disease in the US during spring 2020, the relationship between COVID-19 disease and mortality during the exponential phase was modified by demographic and socioeconomic characteristics of the affected populations. Elderly, males, socioeconomically disadvantaged communities of color and those living in urban centers displayed increased susceptibility to COVID-19 disease and mortality. This indicated that targeted intervention to early detect and mitigate new COVID-19 outbreaks including communication to increase awareness, masks distribution, regular testing and reliable tracking may be required for socioeconomically disadvantaged communities of color and states with higher prevalence of racial and ethnic minorities.
Acknowledgments
Funding: None.
Footnote
Reporting Checklist: The authors have completed the MDAR checklist. Available at http://dx.doi.org/10.21037/jphe-20-96
Peer Review File: Available at http://dx.doi.org/10.21037/jphe-20-96
Conflicts of Interest: All authors have completed the ICMJE uniform disclosure form (available at http://dx.doi.org/10.21037/jphe-20-96). The authors have no conflicts of interest to declare.
Ethical Statement: The authors are accountable for all aspects of the work in ensuring that questions related to the accuracy or integrity of any part of the work are appropriately investigated and resolved. The study was conducted using publicly available data. The study was conducted in accordance with the Declaration of Helsinki (as revised in 2013).
Open Access Statement: This is an Open Access article distributed in accordance with the Creative Commons Attribution-NonCommercial-NoDerivs 4.0 International License (CC BY-NC-ND 4.0), which permits the non-commercial replication and distribution of the article with the strict proviso that no changes or edits are made and the original work is properly cited (including links to both the formal publication through the relevant DOI and the license). See: https://creativecommons.org/licenses/by-nc-nd/4.0/.
References
- Huang C, Wan Y, Li X, et al. Clinical features of patients infected with 2019 novel coronavirus in Wuhan, China. Lancet 2020;395:497-506. [Crossref] [PubMed]
- World Health Organization. Novel coronavirus (2019-nCoV). Situation report 100. Geneva, Switzerland: World Health Organization; 2020. Available online: https://www.who.int/docs/default-source/coronaviruse/situation-reports/20200429-sitrep-100-covid-19.pdf?sfvrsn=bbfbf3d1_2
- World Health Organization. Novel coronavirus (2019-nCoV). Situation report 28. Geneva, Switzerland: World Health Organization; 2020. Available online: https://www.who.int/docs/default-source/coronaviruse/situation-reports/20200217-sitrep-28-covid-19.pdf?sfvrsn=a19cf2ad_2
- Holshue ML, DeBolt C, Lindquist S, et al. First case of 2019 novel coronavirus in the United States. New Engl J Med 2020;382:929-36. [Crossref] [PubMed]
- Wang W, Tang J, Wei F. Updated understanding of the outbreak of 2019 novel coronavirus (2019-nCoV) in Wuhan, China. J Med Virol 2020;92:441-7. [Crossref] [PubMed]
- Xu Z, Shi L, Wang Y, et al. Pathological findings of COVID-19 associated with acute respiratory distress syndrome. Lancet Respir Med 2020;8:420-2. [Crossref] [PubMed]
- Chung M, Bernheim A, Mei X, et al. CT Imaging features of 2019 novel coronavirus (2019-nCoV). Radiology 2020;295:202-7. [Crossref] [PubMed]
- Bernheim A, Mei X, Huang M, et al. Chest CT findings in coronavirus disease-19 (COVID-19): relationship to duration of infection. Radiology 2020;295:200463 [Crossref] [PubMed]
- Grasselli G, Zangrillo A, Zanella A, et al. Baseline characteristics and outcomes of 1591 patients infected with SARS-CoV-2 admitted to ICUs of the Lombardy region, Italy. JAMA 2020;323:1574-81. [Crossref] [PubMed]
- Richardson S, Hirsch JS, Narasimhan M, et al. Presenting characteristics, comorbidities, and outcomes among 5700 patients hospitalized with COVID-19 in the New York City area. JAMA 2020;323:2052-9. [Crossref] [PubMed]
- Zhou F, Yu T, Du R, et al. Clinical course and risk factors for mortality of adult inpatients with COVID-19 in Wuhan, China: a retrospective cohort study. Lancet 2020;395:1054-62. [Crossref] [PubMed]
- Yancy CW. COVID-19 and African Americans. JAMA 2020;323:1891-2. [Crossref] [PubMed]
- Mahajan UV, Larkins-Pettigrew M. Racial demographics and COVID-19 confirmed cases and deaths: a correlational analysis of 2886 US counties. J Public Health (Oxf) 2020;42:445-7. [Crossref] [PubMed]
- Garg S, Kim L, Whitaker M, et al. Hospitalization rates and characteristics of patients hospitalized with laboratory-confirmed coronavirus disease 2019 - COVID-NET, 14 States, March 1-30, 2020. MMWR Morb Mortal Wkly Rep 2020;69:458-64. [Crossref] [PubMed]
- Meddings JB, Scott RB, Fick GH. Analysis and comparison of sigmoidal curves: application to dose-response data. Am J Physiol 1989;257:G982-G989. [PubMed]
- Ritz C, Baty F, Streibig JC, et al. Dose-response analysis using R. PLoS One 2015;10:e0146021 [Crossref] [PubMed]
- Tsoularis A, Wallace J. Analysis of logistic growth models. Math Biosci 2002;179:21-55. [Crossref] [PubMed]
- Gao Y, Zhang Z, Yao W, et al. Forecasting the cumulative number of COVID-19 deaths in China: a Boltzmann function-based modeling study. Infect Control Hosp Epidemiol 2020;41:841-3. [Crossref] [PubMed]
- Melo GC, Araújo Neto RA, Araújo KCGM. Forecasting the rate of cumulative cases of COVID-19 infection in Northeast Brazil: a Boltzmann function-based modeling study. Cad Saude Publica 2020;36:e00105720 [Crossref] [PubMed]
- Dong E, Du H, Gardner L. An interactive web-based dashboard to track COVID-19 in real time. Lancet Infect Dis 2020;20:533-4. [Crossref] [PubMed]
- Seber GAF. Nonlinear Regression Models. In: The Linear Model and Hypothesis. Springer Series in Statistics. Springer, Cham, 2015.
- U.S. Census Bureau. (2020) Our nation, in numbers. Available online: https://usafacts.org/data/topics/people-society/population-and-demographics/population-data/population/ (accessed on May 31, 2020).
- Anselin L, Syabri I, Kho Y. GeoDa: An Introduction to Spatial Data Analysis. Geogr Anal 2006;38:5-22. [Crossref]
- Mathur G, Mathur S. Antibody Testing for COVID-19. Am J Clin Pathol 2020;154:1-3. [Crossref] [PubMed]
- Sood N, Simon P, Ebner P, et al. Seroprevalence of SARS-CoV-2-Specific antibodies among adults in Los Angeles County, California, on April 10-11, 2020. JAMA 2020;323:2425-7. [Crossref] [PubMed]
- Souch JM, Cossman JS. A commentary on rural-urban disparities in COVID-19 testing rates per 100,000 and risk factors. J Rural Health 2020; [Crossref] [PubMed]
- Jarman MP, Castillo RC, Carlini AR, et al. Rural risk: Geographic disparities in trauma mortality. Surgery 2016;160:1551-9. [Crossref] [PubMed]
- Pew Research Center, May 2018, “What Unites and Divides Urban, Suburban and Rural Communities”. Available online: https://www.pewsocialtrends.org/2018/05/22/what-unites-and-divides-urban-suburban-and-rural-communities/
- Kinross P, Suetens C, Gomes Dias J, et al. Rapidly increasing cumulative incidence of coronavirus disease (COVID-19) in the European Union/European Economic Area and the United Kingdom, 1 January to 15 March 2020. Eur Commun Dis Bull 2020;25:2000285.
Cite this article as: Velazquez K, Scott-Hansen A, Chalbot MCG, Kavouras IG. Assessment of state socioeconomic and demographic disparities on coronavirus-2019 mortality during the exponential increase period in the US. J Public Health Emerg 2021;5:14.