The evolution and effects of mobile health (mHealth) intervention on weight management among healthy overweight/obese populations in China: a systematic review and meta-analysis
Introduction
Overweight or obesity has become a serious public health problem around the globe; more than 1.9 billion adults (39%) worldwide were overweight, among whom over 650 million adults (13%) were obese in 2016 (1). Obesity not only brings great inconvenience to body movement, but also associates with increased risks of chronic diseases such as diabetes, high blood pressure, heart disease, and cancers, and even premature death (1). Developing countries typically have lower rates of obesity than developed countries (2); however, globalization and economic development have contributed to the rapid increase of obesity prevalence in developing countries, with no exception in China (3). A national study showed that more than half of Chinese adults were overweight or obese in 2015–2019, which has risen significantly since 1992 when 20% of adults were overweight or obese based on the Chinese criteria (4). This situation can impose an enormous health and economic burden on Chinese people and hinder the sustainable development of the society as well (5).
A considerable number of studies have already demonstrated that adopting and maintaining healthy lifestyle like eating healthy diet and increasing physical activities can be an effective non-pharmacological approach to lose excessive body weight (BW) and modify the risk factors for obesity (6-8). However, it is very difficult for many people who are overweight or obese to change their lifestyle and maintain weight loss by simply knowing the idea (9). Thus, it is crucial to identify a cost-effective strategy that can reach the large target population and facilitate healthy lifestyle adoption and weight management among people with obesity in China.
The exponential growth and wide adoption of mobile technology worldwide in the past decades has led researchers to explore the potential of mHealth interventions using mobile phones as a service delivery platform to prevent and treat obesity among large populations (10,11). To date, several systematic reviews on the efficacy of using mobile apps on lifestyle change and weight management have concluded that incorporating apps can lead to the notable success in weight reduction, physical activity increase, and obesity control (11-15). However, majority of studies conducted in western countries. It is still lack of understanding of how the development of mHealth intervention in weight management in China. Even some reviewed effects of mHealth intervention among Chinese population, most of them only examined the overall effectiveness of mHealth interventions, and did not include subgroup analyses based on mHealth intervention modalities (16). In previous studies, different mHealth intervention modalities (like Short Message Services, phone calls, mobile apps, wearable devices) were considered altogether, and there was a lack of adequate evidence to distinguish the effects of specific features and mHealth modalities on weight management, especially among healthy overweight/obese population, which is a huge population in China (10-16).
This present study aims to (I) describe the evolutionary history of mHealth interventions in weight management in China, and (II) examine the overall and subgroup (traditional vs. intelligent mHealth intervention) effects of mHealth interventions on weight management among healthy overweight/obese populations in China. The findings from this study will enhance our understanding of the development of mHealth interventions in weight management among Chinese population, and provide evidence for implementing and scaling up mHealth interventions as a potential approach to reach a larger population and reverse the obesity crisis in China.
Methods
This present study was conducted and reported following the Preferred Reporting Items for Systematic Reviews and Meta-Analyses (PRISMA) Statement (2020) (available at https://jphe.amegroups.com/article/view/10.21037/jphe-22-54/rc) and followed the predetermined methods documented in a protocol, registered in the International Prospective Register of Systematic Reviews (PROSPERO; registration number: CRD42022343293) (17).
Data sources and search strategy
A total of seven electronic databases [PubMed, MEDLINE (Ovid), Web of Science (Clarivate), Embase (Elsevier), WANFANG, CINAHL Complete (EBSCO) and Google Scholar] were systematically searched for studies published in English or Chinese from June 1 to July 31, 2021. Search terms included combination, truncations, and synonym of the following: (I) Chinese adults, (II) obesity, and (III) mHealth intervention modalities (mobile phone or personal digital assistant or text messaging or mobile apps). Chinese search terms included (I) 肥胖 or 超重, (II) 移动智能干预 (手机 or个人数字助理 or 移动设备 or 短信 or移动应用程序). The search was independently conducted by two reviewers (MC and XP). Search results across databases were merged using reference management software Endnote 20 (Camelot UK Bidco Limited, Clarivate Analytics, United Kingdom), and duplicate records of the same study were removed. Study selection followed the process described in the Cochrane Handbook of Systematic Reviews and PRISMA statements. Two reviewers independently screened titles and abstracts to identify studies that met the inclusion criteria described above. Any disagreements were discussed and resolved by consensus and a third reviewer was consulted in the case of unresolved disagreement.
Eligibility criteria
Studies were eligible for review if the study met the following criteria: (I) randomized control trials (RCTs) or quasi-experimental studies that used phone-based mHealth interventions for weight management and/or healthy lifestyle adoption and maintenance in any settings; (II) a comparator group of participants receiving standard care (no mHealth intervention); (III) participants were healthy people who were overweight (BMI >24 kg/m2) or obese (BMI >28 kg/m2) in China; and (IV) studies reported weight-related measures such as body weight (BW), body mass index (BMI), waist circumference (WC), and/or body fat percentage (BFP) as primary outcomes. Studies were excluded from review if (I) the study intervention included other treatment components (e.g., medications, surgery) besides mHealth intervention; (II) the study did not report the effects of mHealth interventions on BW outcomes of interest; (III) participants had other illness and conditions other than overweight/obesity (e.g., heart disease and stroke, diabetes, cancers, major psychiatric or cognitive condition, pregnancy, etc.); (IV) studies used non-intervention designs (such as case-control studies, cohort studies, cross-sectional studies, case reports and experiences, theory research, and reviews); (V) the study did not conduct among population of interest, or (VI) full text were not available.
Data extraction
Two authors (XP and MC) independently extracted key information from the reviewed articles using a predesigned electronic data extraction table based on PRISMA statement, including (I) general study information: study ID, authors, year of publication, and region of study; (II) study characteristics (study design and sample size); (III) participant characteristics (age, gender, inclusive criteria); (IV) intervention characteristics (intervention type and duration), and (V) weight-related outcomes [BW (kg), BMI (kg/m2), WC (cm), and/or BFP (%)]. The mean and standard deviation (SD) of the outcome measures were extracted to conduct a meta-analysis. Extracted results were compared, and discrepancies were resolved by discussion and consensus.
Study quality assessment
The risk of bias was evaluated in accordance with the Risk of Bias 2 (RoB 2) tool from the Cochrane Handbook for Systematic Reviews of Intervention, considering random sequence generation, allocation concealment, blinding of participants and personnel, blinding of outcome assessment, incomplete outcome data, and selective reporting (18). Each criterion was categorized as low risk, unclear risk, or high risk. Two reviewers independently referred to the instructions and made judgement. The differences between the two were discussed until a consensus decision was reached.
Statistical analysis
A meta-analysis was performed using Cochrane’s Review Manager (RevMan 5.3) (The Nordic Cochrane Centre, Copenhagen, Denmark) to synthesize the overall effect sizes of mHealth interventions on weight-related outcomes (BMI, BW, WC, and/or BFP). Subgroup analysis was also conducted to evaluate and compare the effect sizes of the intelligent and traditional mHealth interventions, as well as the four sub-types of intelligent mHealth interventions (including social media-based, self-management-based, website-based, and wearable devices-based interventions). A random-effect model was used to control for heterogeneity in the intervention effects caused by differences in study populations, interventions received, follow-up length, and other factors between studies (18,19). Heterogeneity was identified by visual inspection of the forest plots and statistically examined with the Higgins I2 test, in which values >75% were considered as highly heterogeneous, 25%<I2≤75% as medium heterogenous, and ≤25% as low heterogeneous (18).
The pooled effect sizes were described as weighted mean differences (WMDs) and 95% confidence intervals (CIs), and statistical significance was considered at a P value <0.05. The WMDs between the experimental and control groups and 95% CIs were estimated using the outcome measure mean change from baseline to the end of the intervention, standard deviation (SD), and total number of participants for each study. Some studies provided the average value of the outcome measures but did not provide the mean or SD. We converted them to mean and SD for consistency. For instance, The SEs and CIs were converted to SDs using appropriate formulas. If a study reported median and interquartile range (IQR), they were converted to mean and SD through mean = median and SD = IQR/1.35 by assuming the overall distribution of these study data does not relevantly deviate from normal distribution (20). For studies without reported SD change between baseline and the end of the intervention, SDs were calculated using the following equation:
Where SDpre corresponds to the SD at baseline, SDpost corresponds to the SD at the end of intervention, and ρ is the correlation coefficient for correlations between measurements taken at baseline and at the end of the intervention. Similar to other studies that implemented valid meta-analysis, we assigned ρ to equal 0.5 (21,22).
Results
A total of 7,610 studies were yielded after the systematic search through the seven online databases, 1,530 of which were excluded because of duplication. After the review of titles and abstracts, 5,940 studies were further excluded for non-related topic, ineligible study design, or not population of interest. Finally, 140 studies underwent full-text review. However, 127 studies were then excluded for not meeting the inclusion criteria mentioned previously. Therefore, a total of 13 studies were included in the final review and meta-analysis (23-35). The PRISMA flow diagram is shown in Figure 1.
Study characteristics
The key study characteristics are summarized in Table 1. All the included studies were published between 2014 and 2021, among which eleven were RCTs (23-27,29-33,35) and two were quasi-experimental studies (28,34). A total of 1,845 participants who were overweight/obese were included in the studies, and the sample size of the reviewed studies varied from 25 to 516. Majority of the studies used the Chinese standard to define overweight and obese (overweight as BMI >24 kg/m2, and obese as BMI >28 kg/m2). Among the 13 studies, two studies examined the effects of mHealth intervention among adolescent population (32,35), nine studies among young and middle-aged adults (23,25-31,33), and two studies among older adults (24,34). The number of participants in experimental and control group in each study were balanced. The percentage of female participants in the reviewed studies ranged from 40% to 70%. The studies were conducted in Beijing (n=2), Shandong (n=2), Zhejiang (n=1), Hong Kong (n=2), Tianjin (n=1), Anhui (n=1), Jiangsu (n=1), Jiangxi (n=1), Guangdong (n=1), and Fujian (n=1) provinces. The mHealth intervention modalities in the reviewed studies varied from traditional interventions like SMS and phone calls (23,24) to intelligent interventions like mobile apps, website-based, and wearable device (25-35). The average duration of the interventions was about 8 months (ranged from 2 to 24 months). Among the 13 studies, 5 reported BW as weight outcome (23,31,33-35), 12 reported BMI (23-34), 7 reported WC (23-25,30-32,34), and 6 reported BFP (23,30-34).
Table 1
Study ID | Author, year | Region | Study design | Sample size | Age, years (mean) | Female (%) | Weight-related inclusive criteria | Type of mHealth services | Duration of intervention | Key outcomes |
---|---|---|---|---|---|---|---|---|---|---|
1 | Lin et al., 2014 (23) | Beijing | RCT | 123 | 38.2 | 60 | Overweight or obese adults (BMI ≥24) | Traditional (SMS and phone calls) | 6 months | BW, BMI, WC, BFP |
2 | Zhang, 2018 (24) | Nanjing, Jiangsu | RCT | 516 | I: 66.7; C: 66.8 | 53 | Adults with BMI ≥28 kg/m2 | Traditional (phone call) | 24 months | BMI, WC |
3 | Li et al., 2016 (25) | Linyi, Shandong | RCT | 100 | 39.7 | 53 | Adults with BMI ≥24 kg/m2 | Intelligent (social media-based) | 3 months + 3-month follow-up | BMI, WC |
4 | Zhou, 2020 (26) | Shaoxing, Zhejiang | RCT | 50 | N/A | N/A | Adults with BMI >28 kg/m2 | Intelligent (social media-based) | 2 months | BMI |
5 | Dong et al., 2017 (27) | Weihai, Shandong | RCT | 119 | I: 52.6; C: 51.3 | 49 | Adults with BMI >24.0 kg/m2 and/or WC ≥85 cm (female) or ≥90 cm (male) | Intelligent (social media-based) | 12 months | BMI |
6 | He et al., 2021 (28) | Shenzhen, Guangdong | Quasi-experimental | 446 | I: 38.7; C: 39.5 | 70 | Adults who were overweight (24 kg/m2 ≤ BMI <28 kg/m2), or obese (BMI ≥28 kg/m2) | Intelligent (social media-based) | 12 months | BMI |
7 | Jiao et al., 2017 (29) | Tianjin | RCT | 60 | 46.2 | 50 | Adults with BMI ≥24 kg/m2 | Intelligent (self-management) | 9 months + 6-month follow-up | BMI |
8 | Wang, 2018 (30) | Zhangzhou, Fujian | RCT | 80 | N/A | 50 | Young adults with BMI ≥25 kg/m2 | Intelligent (self-management) | 4 months | BMI, WC, BFP |
9 | Xiao et al., 2020 (31) | Jiangxi | RCT | 100 | I: 42.4; C: 42.5 | 43 | Adults with overweight/obesity | Intelligent (self-management) | 6 months | BW, BMI, WC, BFP |
10 | Abraham et al., 2015 (32) | Hong Kong | RCT | 48 | 14.4 | 40 | Teens with a BMI greater than 95th percentile for age | Intelligent (web-based) | 6 months | BMI, WC, BFP |
11 | Chung et al., 2014 (33) | Hong Kong | RCT | 60 | 37.4 | 63 | Adults with BMI ≥25 kg/m2 | Intelligent (web-based) | 3 months | BW, BMI, BFP |
12 | Zhou, 2016 (34) | Anhui | Quasi-experimental | 25 | N/A | N/A | adults who were overweight or obese | Intelligent (wearable device) | 2.5 months | BW, WC, BMI, BFP |
13 | Li et al., 2020 (35) | Beijing | RCT | 118 | 14.5 | 49 | Teens with BMI ≥28 kg/m2 | Intelligent (wearable device) | 3 months + 3-month follow-up | BW |
RCT, randomized control trial; SMS, short message service; BW, body weight; BMI, body mass index; WC, waist circumference; BFP, body fat percentage; I, Intervention group; C, Control group.
Assessment of the risk of bias
The overview of the risk of bias for the included studies is presented in Figure 2. All the studies were rated low risk of attrition bias and reporting bias. Majority of the studies were considered as low risks of detection bias. The Li et al. study [2020] was rated high risk of detection bias due to the self-reported weight outcomes by the participants (35). Almost every reviewed study, except Abraham et al.’s study (32), were rated high risk of performance bias due to not using a blind approach. Of the 13 studies, one study did not use random sequence generation or allocation concealment (28), and one study did not report assignment and allocation related information (34). Some other biases were reported or detected in five studies. In Li et al. study [2016], significantly higher drop-out rate was reported in the control group, compared to the intervention group (47% vs. 12%), and the authors used an intention-to-treat (ITT) analysis to minimize the potential bias (25). In Dong et al. study [2017], the intervention group had higher participation rates than the control group, which might influence the observed difference in the outcomes of interest (27). In He et al. study [2021], the attrition reason mentioned by the participants was that it was hard to follow the intervention plans, which can lead to the intervention effect estimate bias (28). Lastly, in Jiao et al. study [2017], higher compliance rate was reported among the intervention group participants, which can bias the effect estimate in favor of the experimental intervention (29).
Evolution of mHealth interventions in China
China has witnessed immense development in mobile technology in the past few decades. In the 1990s, the Ministry of Posts and Telecommunications of China started the wireless telephone communication in the modern sense and a nationwide network was then developed afterwards (36). The number of cell phones rose from near zero in 1990 to around 100 million in 2001, and to 650 million users in 2009. By this time, these cell phone developed at the advent of 3G networks in China referred to the so-called “feature phone”, which used an embedded operating system and had no features beyond basic calling and messaging (37). Starting from around 2009, China issued new generation phone licenses with faster and better capability to handle the internets and multimedia messages (36). Advanced than feature phones, these cell phones with additional functionalities other than calling and texting were defined as “smartphones”. Smartphones enabled various applications to be installed by the users for multiple use, including networking, online payment, online shopping, video streaming, news and content providing, etc. (36). According to the Newzoo’s Global Mobile Market Report 2022, the smartphone penetration increased from 46.9% to 66% from 2013 to 2021, indicating a dramatic boom in the number of smartphone users in China (38). Thanks to the rapid development of technology and mobile phone services, China has experienced a significant growth in the reach and quality of mHealth services all over the country, with the overall mHealth market value increased from US$241.5 million in 2011 to US$2 billion in 2017 (39).
The rapid development of mobile technology and communication systems provided a great opportunity to develop mobile health (mHealth) interventions to support medical and public health practices in improving population health. Mobile Health interventions often compress the functionalities of mobile devices in Short Message Service (SMS), phone calls, web browsing, mobile applications (apps) supported by mobile phones and wearable devices. The traditional mHealth intervention modalities like SMS and phone call, were usually based on the feature phones and served as a way of reminder, alert, education, and motivation (40,41). The intelligent mHealth intervention modalities like mobile apps and wearable devices, which were often based on smartphones, were considered to be more advanced than the traditional modalities, featured by their multifunctionality and scalability. For instance, WeChat-based apps can efficiently carry the patient physiological parameters to geographically distant locations of the physicians and health professionals. Wearable sensors that collect human physiological parameters, such as blood pressure, pulse rate, and blood oxygen level assisted accurately and timely evaluation on patients’ status. Overall, the mHealth interventions have been proved to effectively improve access to health services, treatment adherence, and change in lifestyle (12,42).
In our review, we have observed a transformation in mHealth service from traditional modalities to intelligent modalities along with the development of mobile technologies. Among the 13 studies published during 2014–2021 in our review, only 2 of them used traditional intervention modalities (23,24), while the rest 11 studies all used intelligent intervention strategies (25-35). This illustrated a trend that an increasing number of intelligent mHealth interventions were employed in treating obesity and weight management along with the explosion of smartphone penetration in China after 2009, while the traditional mHealth intervention modalities like using SMS and phone call seemed to be under lower exposure in either clinical applications or research focuses in recent years in China (41,43). Table 2 summarized the key interventions features among the 13 reviewed studies.
Table 2
Study ID | Author, year | Type of mHealth services | Duration of intervention | Intervention group | Control group | Main findings |
---|---|---|---|---|---|---|
1 | Lin et al., 2014 (23) | Traditional (SMS and phone calls) | 6 months | Three 90-min group sessions, five coaching calls and daily text messages | A brief information session | BW change from baseline (P<0.0001)—I: −1.60±0.28 kg; C: 0.24±0.28 kg |
BMI change from baseline (P<0.0001)—I: −0.61±0.10 kg/m2; C: 0.07±0.11 kg/m2 | ||||||
WC change from baseline (P<0.0001)—I: −2.69±0.43 cm; C: −0.13±0.43 cm | ||||||
BFP change from baseline (P=0.0003)—I: −0.66±0.19; C: 0.36±0.20 | ||||||
2 | Zhang, 2018 (24) | Traditional (phone call) | 24 months | Individualized obesity management, including bi-monthly workshop, self-management log, and follow-up phone calls | No intervention | BMI change from baseline (P=0.000)—I: −2.87±0.94 kg/m2; C: 2.56±4.04 kg/m2 |
WC change from baseline (P=0.038)—I: −1.55±7.72 cm; C: −0.05±8.68 cm | ||||||
3 | Li et al., 2016 (25) | Intelligent (social media-based) | 3 months + 3-month follow-up | Usual outpatient care, daily intensive education sessions and individualized dietary feedback via WeChat for the first 4 weeks, and social media-based education and social networking in the rest weeks | Standard outpatient care | BMI change from baseline (P=0.000)—I: −3.2±2.0 kg/m2; C: −2.1±2.5 kg/m2 |
WC change from baseline (P=0.000)—I: −5.2±3.5 cm; C: −3.1±3.2 cm | ||||||
4 | Zhou, 2020 (26) | Intelligent (social media-based) | 2 months | Weekly nutrition workshop, nutrition and weight control news promotion, weight loss log and competition, and nutrition consultation via WeChat | No intervention | BMI change: I: 32.69±3.75 to 31.78±3.62 kg/m2 (P=0.000); C: 32.88±3.85 to 32.75±3.75 kg/m2 (P=0.261) |
5 | Dong et al., 2017 (27) | Intelligent (social media-based) | 12 months | Usual care, plus education sessions, individualized diet and exercise plans and feedback, goal setting and daily log via WeChat for 12 months | Weekly onsite group education sessions for the first 3 months, with monthly follow-up phone call in the rest 9 months | BMI change (P=0.0000): I: 28.6±3.7 to 24.3±2.8 kg/m2; C: 29.3±3.8 to 27.8±2.9 kg/m2 |
6 | He et al., 2021 (28) | Intelligent (social media-based) | 12 months | Usual care plus grouped with dietitian and health manager, for health consultation and support, goal setting and daily log via WeChat, and using “Tizhong Guanjia” app for exercise management for 12 months | Self-weight management with individualized diet and exercise prescriptions | BMI change (P<0.001): I: 27.93±2.70 to 24.85±2.52 kg/m2; C: 27.82±2.34 to 27.76±2.28 kg/m2 |
7 | Jiao et al., 2017 (29) | Intelligent (self-management) | 9 months + 6-month follow-up | 3-month VLCD + exercise intervention, and 6-month app care by interdisciplinary professional team | 3-month VLCD + exercise intervention, and 6-month outpatient care | BMI change from baseline (P<0.01): I: −4.27±4.10 kg/m2; C: −1.55±2.01 kg/m2 |
8 | Wang, 2018 (30) | Intelligent (self-management) | 4 months | Self-management using KEEP app and WeChat group for 4 months, including regular exercise, weekly training session, goal setting, exercise log, and social networking | No intervention | BMI change (P<0.01)—I: 29.98±4.28 to 26.69±4.02 kg/m2; C: 29.09±4.51 to 28.19±3.54 kg/m2 |
WC change (P<0.05)—I: 98.55±13.08 to 90.37±10.08 cm; C: 97.9±11.77 to 95.15±9.89 cm | ||||||
BFP change (P<0.01)—I: 37.11±4.57 to 30.58±4.38; C: 37.03±5.02 to 36.39±4.94 | ||||||
9 | Xiao et al., 2020 (31) | Intelligent (self-management) | 6 months | Received personalized weight management guidance using “mint health” and “KEEP” apps | Standard outpatient care | BW change (P<0.05)—I: 79.22±9.23 to 71.85±5.22 kg; C: 79.13±9.05 to 74.36±6.57 kg |
BMI change (P<0.01)—I: 27.49±1.51 to 24.13±1.05 kg/m2; C: 27.42±1.48 to 25.85±1.26 kg/m2 | ||||||
WC change (P<0.05)—I: 89.72±7.16 to 83.26±5.18 cm; C: 89.83±7.11 to 85.61±6.46 cm | ||||||
BFP change (P<0.05)—I: 33.15±2.83 to 30.07±1.67; C: 33.11±2.78 to 32.16±2.15 | ||||||
10 | Abraham et al., 2015 (32) | Intelligent (web-based) | 6 months | Usual care together with 12-week internet-based curriculum and semi-personalized cell phone and SMS follow-up over 6 months | Usual care | BMI change—I: 29.3 (IR: 26.7–30.9) to 28.4 (IR: 26.7–31.9) kg/m2; C: 30.1 (IR: 28.4–32.3) to 30.5 (IR: 28.7–32.0) kg/m2 |
WC change—I: 96.6 (IR: 92.2–106.7) to 94.9 (IR: 92.0–100.5) cm; C: 103.0 (IR: 96.1–108.7) to 101.6 (IR: 93.5–107.9) cm | ||||||
BFP change—I: 33.6 (IR: 27.3–42.0) to 31.6 (IR: 26.0–42.4); C: 36.5 (IR: 31.3–41.0) to 37.6 (IR: 30.5–43.6) | ||||||
11 | Chung et al., 2014 (33) | Intelligent (web-based) | 3 months | Dietary log and evaluation using the electronic dietary recording system—the eDietary Intake Portal | No intervention | BW change (P=0.009)—I: 70.0±17.7 to 68.4±17.1 kg; C: 71.7±14.4 to 70.2±13.7 kg |
BMI change (P=0.003)—I: 27.8±4.4 to 27.3±4.2 kg/m2; C: 28.1±2.3 to 28.0±2.6 kg/m2 | ||||||
BFP change (P=0.795)—I: 33.8±6.7 to 33.4±6.5; C: 32.9±6.1 to 33.9±7.1 | ||||||
12 | Zhou, 2016 (34) | Intelligent (wearable device) | 2.5 months | Personalized exercise prescription and regular exercise wearing MIO FUSE and MIO Alpha 2 watches for 10 weeks | No intervention | BW change from baseline (P<0.01)—I: −0.96±0.74 kg; C: 0.72±0.85 kg |
BMI change from baseline (P<0.01)—I: −0.40±0.32 kg/m2; C: 0.28±0.33 kg/m2 | ||||||
WC change from baseline (P<0.01)—I: −2.0±2.83 cm; C: 1.46±2.6 cm | ||||||
BFP change from baseline (P<0.01)—I: −0.01±0.01; C: 0.01±0.01 | ||||||
13 | Li et al., 2020 (35) | Intelligent (wearable device) | 3 months + 3-month follow-up | Usual care plus 3-month remote exercise support via wearable device and “Gudong” exercise app (including personalized exercise plan, monitoring, evaluation and adjustment) | Standard outpatient care | BW change (P<0.01): I: 75.39±5.54 to 66.34±5.31 kg; C: 76.49±5.31 to 72.88±5.44 kg |
Main findings were expressed as mean and SD except for Abraham et al. study [2015]. SMS, Short Message Services; VLCD, very low carbohydrate diet; RCT, randomized control trial; BW, body weight; BMI, body mass index; WC, waist circumference; BFP, body fat percentage; IR, interquartile range; I, Intervention group; C, Control group.
The overall effects of mHealth intervention on weight management
Overall, the pooled effect estimations based on all the reviewed studies showed that the mHealth intervention groups had significant reductions in BW (WMD =−1.84 kg, 95% CI: −1.94, −1.74, I2=0%, P=0.93), BMI (WMD =−1.98 kg/m2, 95% CI: −2.94, −1.03, I2=98%, P<0.00001), WC (WMD =−2.32 cm, 95% CI: −2.83, −1.81, I2=27%, P=0.22), and BFP (WMD =−1.52, 95% CI: −2.31, −0.74, I2=99%, P<0.00001), when compared to the control/usual care groups (Figure 3).
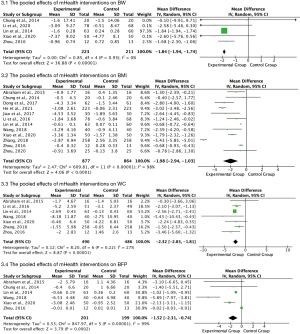
In the following subgroup analysis, we examined and compared the effects of traditional and intelligent mHealth interventions on weight loss among healthy Chinese who were overweight/obese. Moreover, we further categorized intelligent intervention modalities into four sub-types: (I) social media-based (25-28); (II) self-management-based (29-31), (III) web-based (32,33), and (IV) wearable devices (34,35), and examined their effects on weight outcomes respectively.
The effects of traditional mHealth interventions on weight management
Two studies assessed the effects of tradition mHealth interventions using SMS and/or phone calls on weight loss (23,24). Both studies examined BMI and WC changes as weight outcome measures, while only one study also reported BW and BFP changes (23,24). The pooled effect test results showed that participants in the intervention group had significant changes in BW of −1.84 kg (95% CI: −1.94, −1.74), and WC of −2.17 cm (95% CI: −3.17, −1.17), and BFP of −1.02 (95% CI: −1.09, −0.95), while the change in BMI (WMD =−3.05 kg/m2, 95% CI: −7.71, 1.60) was not statistically significant, compared to the control/usual care groups. High to medium heterogeneity was observed between the two studies when examining the pooled effects on BMI (I2=100%, P<0.00001) and WC (I2=73%, P=0.06) (Figure 4).
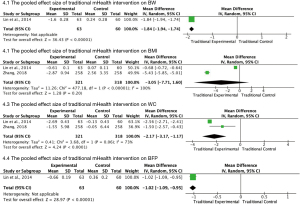
The effects of intelligent mHealth interventions on weight management
Eleven studies assessed the effects of intelligent mHealth interventions on reducing BW, BMI, WC and/or BFP among the healthy overweight/obese participants (25-35). Overall, compared to those in control groups who received no or regular care, participants in the intervention groups experienced significant reductions in their BW (WMD =−2.66 kg, 95% CI: −4.42, −0.89), BMI (WMD =−1.72 kg/m2, 95% CI: −2.48, −0.96), WC (WMD =−2.31 cm, 95% CI: −3.17, −1.45), and BFP (WMD =−2.41, 95% CI: −4.52, −0.31) (Table 3). There was substantial heterogeneity for BFP (I2=92%), BMI (I2=90%) and BW (I2=59%), while very low heterogeneity was observed for WC (I2=4%).
Table 3
Subgroups | BW (kg) | BMI (kg/m2) | WC (cm) | BFP (%) |
---|---|---|---|---|
Intelligent interventions | ||||
Social media-based | N/A | MD: −2.04; 95% CI: (−3.28, −0.80); I2 index: 88%; Z=3.21, P=0.001 | MD: −2.10; 95% CI: (−3.07, −1.13); I2 index: NA; Z=4.23, P<0.0001 | N/A |
Self-management-based | MD: −2.60; 95% CI: (−5.76, 0.56); I2 index: NA; Z=1.61, P=0.11 | MD: −1.90; 95% CI: (−2.39, −1.40); I2 index: 0%; Z=7.55, P<0.00001 | MD: −3.09; 95% CI: (−5.85, −0.33); I2 index: 19%; Z=2.19, P=0.03 | MD: −3.89; 95% CI: (−7.57, −0.22); I2 index: 90%; Z=2.08, P=0.04 |
Web-based | MD: −0.10; 95% CI: (−9.91, 9.71); I2 index: NA; Z=0.02, P=0.98 | MD: −1.12; 95% CI: (−2.09, −0.14); I2 index: 0%; Z=2.25, P=0.02 | MD: −0.30; 95% CI: (−3.66, 3.06); I2 index: NA; Z=0.17, P=0.86 | MD: −2.37; 95% CI: (−5.06, 0.31); I2 index: 0%; Z=1.73, P=0.08 |
Wearable devices | MD: −2.89; 95% CI: (−5.56, −0.22); I2 index: 86%; Z=2.12, P=0.03 | MD: −0.68; 95% CI: (−0.93, −0.43); I2 index: NA; Z=5.23, P<0.00001 | MD: −3.46; 95% CI: (−5.60, −1.32); I2 index: NA; Z=3.18, P=0.001 | MD: −0.02; 95% CI: (−0.03, −0.01); I2 index: NA; Z=5.00, P<0.00001 |
All intelligent interventions | MD: −2.66; 95% CI: (−4.42, −0.89); I2 index: 59%; Z=2.95, P=0.003 | MD: −1.72; 95% CI: (−2.48, −0.96); I2 index: 90%; Z=4.41, P<0.0001 | MD: −2.31; 95% CI: (−3.17, −1.45); I2 index: 4%; Z=5.26, P<0.00001 | MD: −2.41; 95% CI: (−4.52, −0.31); I2 index: 92%; Z=2.25, P=0.02 |
Traditional interventions | ||||
All traditional interventions | MD: −1.84; 95% CI: (−1.94, −1.74); I2 index: NA; Z=36.43, P<0.00001 | MD: −3.05; 95% CI: (−7.71, 1.60); I2 index: 100%; Z=1.28, P=0.20 | MD: −2.17; 95% CI: (−3.17, −1.17); I2 index: 73%; Z=4.24, P<0.0001 | MD: −1.02; 95% CI: (−1.09, −0.95); I2 index: NA; Z=28.97, P<0.00001 |
All mHealth intervention | ||||
All mHealth interventions | MD: −1.84; 95% CI: (−1.94, −1.74); I2 index: 0%; Z=36.88, P<0.00001 | MD: −1.98; 95% CI: (−2.94, −1.03); I2 index: 98%; Z=4.06, P<0.0001 | MD: −2.32; 95% CI: (−2.83, −1.81); I2 index: 27%; Z=8.87, P<0.00001 | MD: −1.52; 95% CI: (−2.31, −0.74); I2 index: 99%; Z=3.79, P=0.0002 |
BW, body weight; BMI, body mass index; WC, waist circumference; BFP, body fat percentage; N/A: not applicable; MD, mean difference.
The effects of subtypes of intelligent mHealth interventions on BW
None of the reviewed studies reported the effects of social media-based intervention on BW reduction. The Xiao et al. study [2020] assessed the effects of self-management intervention on BW change and reported a BW reduction of 2.60 kg (95% CI: −5.76, 0.56) in the intervention group, when compared to the control group (31). One study assessed the effects of web-based intervention on BW and found a BW decrease of 0.10 kg (95% CI: −9.91, 9.71) in the intervention group, compared to the control group (33). Two studies (34,35) assessed the effects of wearable devices and the pooled effects on BW change was significant (WMD =−2.89 kg, 95% CI: −5.56, −0.22) among the participants in the intervention group, compared to those in the control group. High heterogeneity was observed between the two studies (I2=86%, P=0.008). Among the four intelligent mHealth intervention subtypes, the wearable device intervention stood out for contributing to the most significant BW reduction (Figure 5).

The effects of subtypes of intelligent mHealth interventions on BMI
Four studies (25-28) assessed the effects of social media-based intervention, and the pooled effect on BMI was −2.04 kg/m2 (95% CI: −3.28, −0.80) among the participants in the intervention groups, compared to those in the control groups, with a high heterogeneity (I2=88%, P<0.0001). Three studies (29-31) examined the effects of self-management interventions and showed a pooled effect on BMI of −1.90 kg/m2 (95% CI: −2.39, −1.40) among the intervention groups when compared to the control group, with no evidence of heterogeneity (I2=0%, P=0.58). The pooled effects of web-based intervention on BMI based on two studies (32,33) was −1.12 kg/m2 (95% CI: −2.09, −0.14) in the intervention groups, compared to the control groups, with no evidence of heterogeneity (I2=0%, P=0.47). Only one study reported the effect of using wearable devices on BMI of −0.68 kg/m2 (95% CI: −0.93, −0.43) among participants in the intervention group when compared to the control group (34). Among the four subtypes of intelligent intervention modalities, social-media-based mHealth stood out for contributing to the most significant BMI reduction (Figure 6).
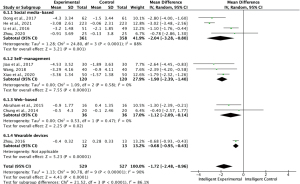
The effects of subtypes of intelligent mHealth interventions on WC
Li et al. study [2016] evaluated the effect of social media-based intervention on WC and reported a significant change of −2.10 cm (95% CI: −3.07, −1.13) in the intervention group, compared to the control groups (25). Two studies (30,31) assessed the effects of self-management interventions on WC reduction and the pooled effect was −3.09 cm (95% CI: −5.85, −0.33) among the intervention groups when compared to the control groups, with a low heterogeneity (I2=19%, P=0.27). Abraham et al. study reported the effect of web-based intervention on WC of −0.30 cm (95% CI: −3.66, 3.06) in the intervention group; however, it was not statistically significant (32). Zhou’s study using the wearable device as the intervention modality found that the intervention group had a significant reduction in WC, compared to the control group (WMD =−3.46 cm, 95% CI: −5.60, −1.32) (34). Among the four intelligent subtypes, wearable device intervention stood out for contributing to the most significant WC reduction (Figure 7).
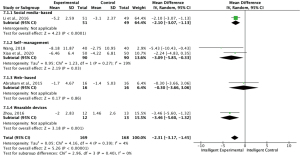
The effects of subtypes of intelligent mHealth interventions on BFP
None of the reviewed studies examined the effects of social media-based intervention on BFP reduction. The pooled effect of the self-management intervention on BFP based on two reviewed studies (30,31) was −3.89 (95% CI: −7.57, −0.22) among the intervention groups, with a high heterogeneity (I2=90%, P=0.001). The pooled effect of web-based interventions on BFP based on two reviewed studies (32,33) was −2.37 (95% CI: −5.06, 0.31) among the intervention groups, with no evidence of heterogeneity (I2=0%, P=0.54). One study (34) assessed the effect of wearable device and found a significant decrease in BFP among the participants in the intervention group when compared to the control group (WMD =−0.02, 95% CI: −0.03, −0.01). Among the four intelligent intervention subtypes, self-management modality appeared to be superior to other subtypes, in terms of reducing BFP among healthy overweight/obese population (Figure 8).
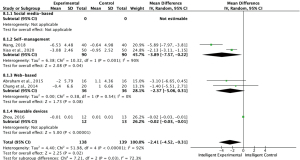
Comparison of the effects of the traditional and intelligent interventions on weight outcomes
BW
Only one study (23) reported the effect of the mHealth interventions using traditional modality-daily SMS and coaching phone calls on BW and reported a significant reduction in BW of −1.84 kg (95% CI: −1.94, −1.74). The pooled effect of intelligent interventions on BW was −2.66 kg (95% CI: −4.42, −0.89), with a medium heterogeneity among the four studies (I2=59%, P=0.06) (Table 3). The effect size of the intelligent interventions on BW reduction was 0.82kg larger than that of the traditional interventions.
BMI
The pooled effect of the traditional mHealth interventions on BMI based on two reviewed studies (23,24) was not statistically significant (WMD =−3.05 kg/m2, 95% CI: −7.71, 1.60), with a high heterogeneity (I2=100%, P<0.00001). On the other hand, the pooled effect of the intelligent mHealth interventions on BMI was estimated as −1.72 kg/m2 (95% CI: −2.48, −0.96) among the intervention when compared to the control groups, with high heterogeneity observed among the studies (I2=90%, P<0.00001) (Table 3). According to the results, no valid comparison conclusion can be drawn between the two types of interventions, because the traditional mHealth interventions showed no statistically significant effect on decreasing BMI, although its pooled effect size was larger than that of the intelligent interventions.
WC
The pooled effect of the traditional mHealth interventions on reducing WC was significant among the intervention groups, compared with the control group (WMD =−2.17 cm, 95% CI: −3.17, −1.17), with a medium heterogeneity (I2=73%, P=0.06). On the other hand, the pooled effect test result showed that participants receiving the intelligent mHealth interventions had a significant decrease in WC, compared to the control groups (WMD =−2.31 cm, 95% CI: −3.17, −1.45), with very low heterogeneity (I2=4%, P=0.39) (Table 3). The intelligent interventions appeared to be slightly superior to the traditional interventions in terms of reducing WC.
BFP
Lin et al. study [2014] reported a significant effect of the traditional mHealth interventions on BFP of −1.02 (95% CI: −1.09, −0.95) (23). The pooled effect of intelligent interventions on BFP change was −2.41 (95% CI: −4.52, −0.31), with a high heterogeneity among the five reviewed studies (I2=92%, P<0.00001). The effect size of the intelligent interventions on BFP reduction was 1.39 larger than that of the traditional interventions (Table 3).
Discussion
This systematic review and meta-analysis study described the evolution of the mHealth interventions in weight management in China and evaluated the overall and subgroup effects of mHealth interventions on weight loss among healthy overweight/obese Chinese population. In the study, we observed a transformation of the mHealth intervention modalities from traditional to intelligent interventions along with the development of mobile technology and communication systems during the reviewed period 2014–2021 in China. There was significantly higher volume of mHealth interventions based on smartphone platform to deliver weight management care, than that of traditional interventions based on feature phones in recent years. This trend can be explained by the rapid increase of smartphones ownerships in China after 2009, and the advantages of intelligent intervention modalities compared to the traditional ones. Traditional ways of managing the patients’ weights were limited to sending text messages or delivering phone calls as reminders for regular clinical follow-up visit, medicine taking, and education delivery, while the intelligent mHealth interventions optimized the information delivery system by providing simultaneous communication channel such as WeChat friends, group chat to ensure sufficient and timely contact between patients and health care workers. Advanced features such as providing exercise instructions, daily recommendations of dietary and physical activities, and personal calorie consumptions further expanded the function of mobile apps on weight management.
The meta-analysis results showed that the intelligent mHealth interventions were effective in reducing all the four weight-related outcomes (BW, BMI, WC, and BFP). Previous studies reviewed effects of mHealth intervention on weight loss, and reported an average pooled intervention effects of 1.49 kg BW loss and 0.56 kg/m2 BMI reduction, compared to the control groups (42,44,45). Our study results, in general, aligned with the previous studies, but found larger pooled effect sizes of mHealth interventions on both BW and BMI reductions (1.84 kg and 1.98 kg/m2, respectively) among the healthy overweight/obese Chinese population. Future studies are needed to validate the findings from the present study.
The comparison results between the traditional and intelligent mHealth interventions showed that the intelligent interventions appeared to be more effective in weight loss, especially in reducing BW (2.66 vs. 1.84 kg), WC (2.31 vs. 2.17 cm) and BFP (2.41 vs. 1.02). When further comparing the four subtypes of the intelligent mHealth interventions, wearable device-based interventions were found to be the most effective in reducing BW and WC, while the social-media-based interventions seemed to yield more reduction in BMI and the self-management-based interventions appeared to be more effective in reducing BFP among healthy overweight/obese population in China.
The effectiveness of the wearable device-based interventions in weight loss, especially in BW and WC reduction, may be attributable to the unique features introduced by the wearable devices. Compared to the conventional exercise interventions, the wearable devices and their supporting systems allowed real-time health statistics monitoring, provided more personalized and effective exercise interval, training intensity and optimal duration of exercise training. These features not only made it easier for the participants to follow and complete the exercise plans, but also allow the participants to self-monitor and adjust exercise settings based on their own situations, which could increase their confidence and compliance with the exercise plans and achieve the short- and long-term weight management goals. Moreover, in Li et al. study, there was a daily ranking that participants could watch the daily exercise completed by other participants, which stimulated the participants into initiating and completing exercise, improved their self-regulation and compliance with the intervention plans.
The social-media-based interventions in the reviewed studies were majorly using WeChat as the media platform. WeChat is a leading communication tool dominating the Chinese social life with over 600 million citizens as its users (46). The common strategies used in the social-media-based interventions included sending group massages that were beneficial to weight management, providing one-on-one professional feedback from experts, and creating “the community effect”. The educational messages made a subtle but significant impact on establishing correct health maintaining values to the patients. The highly personalized weight management advice provided by medical experts was an essential booster for patients to make consistent efforts in the correct direction. When participants in the same group chat, WeChat applet or official account saw each other’s progress like exercising data and weight loss records, this generated the “the community effect” to motivate and encourage the participants to perform better (like do more exercise and win a higher rank in step count competition), and make them feel that they are supported by the whole community, and they are not alone in this weight loss journey. On the other hand, the effectiveness of the self-management intervention in weight management may be attributable to its tailoring to the individuals by using the users’ personal data and generating individualized plans for users’ everyday physical activities and dietary habits in different phases through algorithms. The algorithms were usually designed by multiple medical experts to give advice regarding different situations provided by the users.
With the fastest growing ageing populations and the increasing prevalence of obesity among the elderly population in China, the development of effective, tailored interventions for this population is needed (47). However, although mHealth interventions based on smartphone platform could be a more effective approach in weight management, there were significant concerns about the acceptance and adoption of such interventions among the elderly populations. According to Qi’s study [2021], although there was 65.5% overall rate of mobile phone use among the Chinese elderly people aged 60 and above, only 14.3% of them used smartphone (48). This means that the elderly population could only have access to the basic or traditional functions of a mobile phone to receive text messages and phone calls. They may not be able to experience the “advantages” the intelligent services bring as they may have limited or no access to smartphones or it is challenging for them to use the smartphones even they are equipped with one. Moreover, according to our review, only a few of the studies (24,34) have experimented the mHealth interventions among elderly population in China, and the results revealed that traditional interventions like phone calls seemed to yield more reduction in BMI, compared to the intelligent interventions that used wearable devices among this population (−2.87±0.94 vs. −0.40±0.32 kg/m2). More studies are needed to validate the findings from the current study and further examine the barriers that elderly population may encounter in adopting and using the intelligent services for weight management.
To the authors’ knowledge, this study is among the first few to review the development of the mHealth intervention on weight management in China, examine the effects of mHealth interventions on weight loss among healthy overweight/obese Chinese population, and distinguish the effects of traditional and intelligent mHealth interventions on different weight outcomes. There are several limitations in this study. First, the risks of bias presented in some of the reviewed studies need to be noted when interpreting the findings from this review. For instance, majority of the reviewed studies did not blind the participants and personnel, which may lead to potential bias. However, we should note that the main reason of this failure was the impracticality of blinding interventions given the nature of the intervention. Moreover, since the outcome measures in the reviewed studies were biomarkers like BMI and BFP, which were relatively objective, it is less likely that the results of the studies were compromised. Second, the findings of our study should be interpreted with caution considering the high heterogeneity among the reviewed studies. For instance, the I2 value was 88% among the four studies that were used to examine the pooled effects of social media-based mHealth interventions on BMI reduction (25-28). The high heterogeneity could be caused by the significant variations of study characteristics like study design (three RCTs and one quasi-experimental study), sample sizes (ranged from 50 to 446), geolocation (two conduced in Northern China, and two in the South), gender (female participants in the studies ranged from 49% to 70%), and intervention activities and intervention durations (ranged from 2 to 12 months) across the four studies. Third, each subgroup only contained a very small number of studies, which can limit the accuracy and generatability of the findings from the subgroup analysis. We therefore propose larger-scale RCTs with long duration and diverse age groups to confirm the findings from this review. Lastly, there was a large discrepancy in the number of the traditional and intelligent intervention studies in this review, which could cause bias and lead to inaccuracy of the findings from the current study. Moreover, no study in this review examined the effects of traditional mHealth interventions on BW or BFP change, which limited our ability to comprehensively evaluate and compare the effects of intelligent and traditional interventions on all the four weight-related outcomes. More RCTs that examine the effects of traditional interventions on different weight outcomes among Chinese population are needed in future.
Conclusions
There was a transformation of the weight management mHealth intervention modalities in China along with the development of mobile technology and communication system in recent years. The mHealth interventions, especially those using intelligent modalities like social media and self-management apps, appeared to be promising in reaching larger target populations, yielding ideal weight loss results, and reversing the obesity crisis in China. Future studies are needed to validate the findings from the current study and examine the effects of mHealth in weight management among vulnerable and elderly population.
Acknowledgments
The authors would like to thank the Undergraduate Office and Community Health Service Lab at Duke Kunshan University for their support throughout the project.
Funding: None.
Footnote
Provenance and Peer Review: This article was commissioned by the Guest Editors (Mellissa Withers and Mary Schooling) for the series “Global Urban Health: Findings from the 2021 APRU Global Health” published in Journal of Public Health and Emergency. The article has undergone external peer review.
Reporting Checklist: The authors have completed the PRISMA reporting checklist. Available at https://jphe.amegroups.com/article/view/10.21037/jphe-22-54/rc
Data Sharing Statement: Available at https://jphe.amegroups.com/article/view/10.21037/jphe-22-54/dss
Conflicts of Interest: Both authors have completed the ICMJE uniform disclosure form (available at https://jphe.amegroups.com/article/view/10.21037/jphe-22-54/coif). The series “Global Urban Health: Findings from the 2021 APRU Global Health” was commissioned by the editorial office without any funding or sponsorship. The authors have no other conflicts of interest to declare.
Ethical Statement: The authors are accountable for all aspects of the work in ensuring that questions related to the accuracy or integrity of any part of the work are appropriately investigated and resolved.
Open Access Statement: This is an Open Access article distributed in accordance with the Creative Commons Attribution-NonCommercial-NoDerivs 4.0 International License (CC BY-NC-ND 4.0), which permits the non-commercial replication and distribution of the article with the strict proviso that no changes or edits are made and the original work is properly cited (including links to both the formal publication through the relevant DOI and the license). See: https://creativecommons.org/licenses/by-nc-nd/4.0/.
References
- World Health Organization. Obesity and overweight 2022 [updated June 9, 2021; cited 2022 Aug 30]. Available online: https://www.who.int/news-room/fact-sheets/detail/obesity-and-overweight
- World Health Organization. Prevalence of overweight among adults, BMI ≥ 25, age-standardized Estimates by World Bank income group: World Health Organization.; 2021 [updated 2017-09-27; cited 2022 Aug 30]. Available online: https://apps.who.int/gho/data/node.main.A897A?lang=en
- Ng M, Fleming T, Robinson M, et al. Global, regional, and national prevalence of overweight and obesity in children and adults during 1980-2013: a systematic analysis for the Global Burden of Disease Study 2013. Lancet 2014;384:766-81. [Crossref] [PubMed]
- Pan XF, Wang L, Pan A. Epidemiology and determinants of obesity in China. Lancet Diabetes Endocrinol 2021;9:373-92. [Crossref] [PubMed]
- Peng W, Zhang J, Zhou H, et al. The 2022 World Obesity Day and obesity prevention and control efforts in China. Global Health Journal 2022. Available online: https://www.sciencedirect.com/science/article/pii/S2414644722000513
- Franz MJ, Boucher JL, Rutten-Ramos S, et al. Lifestyle weight-loss intervention outcomes in overweight and obese adults with type 2 diabetes: a systematic review and meta-analysis of randomized clinical trials. J Acad Nutr Diet 2015;115:1447-63. [Crossref] [PubMed]
- Wing RRLook AHEAD Research Group. Does Lifestyle Intervention Improve Health of Adults with Overweight/Obesity and Type 2 Diabetes? Findings from the Look AHEAD Randomized Trial. Obesity (Silver Spring) 2021;29:1246-58. [Crossref] [PubMed]
- van Namen M, Prendergast L, Peiris C. Supervised lifestyle intervention for people with metabolic syndrome improves outcomes and reduces individual risk factors of metabolic syndrome: A systematic review and meta-analysis. Metabolism 2019;101:153988. [Crossref] [PubMed]
- Dombrowski SU, Knittle K, Avenell A, et al. Long term maintenance of weight loss with non-surgical interventions in obese adults: systematic review and meta-analyses of randomised controlled trials. BMJ 2014;348:g2646. [Crossref] [PubMed]
- Schoeppe S, Alley S, Van Lippevelde W, et al. Efficacy of interventions that use apps to improve diet, physical activity and sedentary behaviour: a systematic review. Int J Behav Nutr Phys Act 2016;13:127. [Crossref] [PubMed]
- Villinger K, Wahl DR, Boeing H, et al. The effectiveness of app-based mobile interventions on nutrition behaviours and nutrition-related health outcomes: A systematic review and meta-analysis. Obes Rev 2019;20:1465-84. [Crossref] [PubMed]
- Wang Y, Min J, Khuri J, et al. Effectiveness of Mobile Health Interventions on Diabetes and Obesity Treatment and Management: Systematic Review of Systematic Reviews. JMIR Mhealth Uhealth 2020;8:e15400. [Crossref] [PubMed]
- Cavero-Redondo I, Martinez-Vizcaino V, Fernandez-Rodriguez R, et al. Effect of Behavioral Weight Management Interventions Using Lifestyle mHealth Self-Monitoring on Weight Loss: A Systematic Review and Meta-Analysis. Nutrients 2020;12:1977. [Crossref] [PubMed]
- Kim HN, Seo K. Smartphone-Based Health Program for Improving Physical Activity and Tackling Obesity for Young Adults: A Systematic Review and Meta-Analysis. Int J Environ Res Public Health 2019;17:15. [Crossref] [PubMed]
- Wu X, Guo X, Zhang Z. The Efficacy of Mobile Phone Apps for Lifestyle Modification in Diabetes: Systematic Review and Meta-Analysis. JMIR Mhealth Uhealth 2019;7:e12297. [Crossref] [PubMed]
- Wei J, Vinnikova A, Lu L, et al. Understanding and Predicting the Adoption of Fitness Mobile Apps: Evidence from China. Health Commun 2021;36:950-61. [Crossref] [PubMed]
- Moher D, Liberati A, Tetzlaff J, et al. Preferred reporting items for systematic reviews and meta-analyses: the PRISMA statement. Ann Intern Med 2009;151:264-9, W64.
- Higgins JP, Thomas J, Chandler J, et al. Cochrane handbook for systematic reviews of interventions: John Wiley & Sons, 2019.
- Borenstein M, Hedges LV, Higgins JP, Rothstein HR. A basic introduction to fixed-effect and random-effects models for meta-analysis. Res Synth Methods 2010;1:97-111. [Crossref] [PubMed]
- Wan X, Wang W, Liu J, et al. Estimating the sample mean and standard deviation from the sample size, median, range and/or interquartile range. BMC Med Res Methodol 2014;14:135. [Crossref] [PubMed]
- Follmann D, Elliott P, Suh I, et al. Variance imputation for overviews of clinical trials with continuous response. J Clin Epidemiol 1992;45:769-73. [Crossref] [PubMed]
- Abrams KR, Gillies CL, Lambert PC. Meta-analysis of heterogeneously reported trials assessing change from baseline. Stat Med 2005;24:3823-44. [Crossref] [PubMed]
- Lin PH, Wang Y, Levine E, et al. A text messaging-assisted randomized lifestyle weight loss clinical trial among overweight adults in Beijing. Obesity (Silver Spring) 2014;22:E29-37. [Crossref] [PubMed]
- Zhang RZ. Analysis on 632the effect of health management of elderly with obesity a community in Nanjing. The East-south University, 2018 [cited 2022 Jun 26].
- Li GC, Nie QL, Meng C, et al. Effect of health education on weight loss of adults 636with obesity using WeChat. Qilu Huli Zazhi 2016;22:21-3.
- Zhou C. Influence of Internet-based nutrition education on weight, cognition, belief and 639 behavior of College Students with obesity. Dazhong Biaozhunhua 2020;63-4.
- Dong CL, Jia RJ, Hou JH. Analysis of intervention effect of 642health management program based on WeChat on high-risk groups with overweight and obese 643diabetes. Zhonghua Jiankang Guanlixue Zazhi 2017;11:531-6.64428.
- He H, Wu WQ, Peng R, et al. Evaluation on the effect of remote 646lifestyle intervention on body weight and related indicators management of people with 647overweight and obesity. Zhonghua Jiankang Guanlixue Zazhi 2021;15:151-7.64829.
- Jiao HL, Liu SH, Zhang Q, et al. Follow-up practice of interactive management of health and weight loss 650by mobile app. Linchuang Huicui 2017;32:400-4.65130.
- Wang SJ. Application of fitness app in self-health management of college students with obesity. Fujian Shangxueyuan Bao 2018;(05):95-100.65431.
- Xiao YP, Zhang CM, Qi M, et al. Application value of "Mint health" combined with "keep" app in personalized weight management. Zhongguo Yixue Chuangxin 2020;17:92-6.
- Abraham AA, Chow WC, So HK, et al. Lifestyle intervention using an internet-based curriculum with cell phone reminders for obese Chinese teens: a randomized controlled study. PLoS One 2015;10:e0125673. [Crossref] [PubMed]
- Chung LM, Law QP, Fong SS, et al. Teledietetics improves weight reduction by modifying eating behavior: a randomized controlled trial. Telemed J E Health 2014;20:55-62. [Crossref] [PubMed]
- Zhou T. Design and research of intelligent health management service system on chronic disease based on mobile terminal. Anhui University, 2016 [cited 2022 Jun].
- Li CP, Zhao HZ, Yang H, et al. Observation on the effect of distant exercise support in weight loss and maintenance of adolescents with obesity. Zhonghua Xiandai Huli Zazhi 2020;26:2759-63.
- Hays AJ. Cell Phones, Smart Phones and Apps in China 2022 [updated May 2022; cited 2022 Aug 30]. Available online: https://factsanddetails.com/china/cat7/sub44/item230.html
- Nagpal P, Lyytinen KJ. Key Actors in the mobile telephone industry: Feature phone years and the rise of Nokia. Nagpal, P, Lyytinen, K. 2013.
- Chen, S. Newzoo Global Mobile Market Report 2021 2021 [cited 2022 Jul 9]. Available online: https://newzoo.com/insights/trend-reports/newzoo-global-mobile-market-report-2021-free-version
- Ray P, Wijaya S, Junxiang Z. mHealth in China: A growing market. Asian Hospital & Healthcare Management 2022.
- Shaw R, Bosworth H. Short message service (SMS) text messaging as an intervention medium for weight loss: A literature review. Health Informatics J 2012;18:235-50. [Crossref] [PubMed]
- Marcolino MS, Oliveira JAQ, D'Agostino M, et al. The Impact of mHealth Interventions: Systematic Review of Systematic Reviews. JMIR Mhealth Uhealth 2018;6:e23. [Crossref] [PubMed]
- Islam MM, Poly TN, Walther BA, et al. Use of Mobile Phone App Interventions to Promote Weight Loss: Meta-Analysis. JMIR Mhealth Uhealth 2020;8:e17039. [Crossref] [PubMed]
- Sahu M, Grover A, Joshi A. Role of mobile phone technology in health education in Asian and African countries: a systematic review. Int J Electron Healthc 2014;7:269-86. [Crossref] [PubMed]
- Park SH, Hwang J, Choi YK. Effect of Mobile Health on Obese Adults: A Systematic Review and Meta-Analysis. Healthc Inform Res 2019;25:12-26. [Crossref] [PubMed]
- Flores Mateo G, Granado-Font E, Ferré-Grau C, et al. Mobile Phone Apps to Promote Weight Loss and Increase Physical Activity: A Systematic Review and Meta-Analysis. J Med Internet Res 2015;17:e253. [Crossref] [PubMed]
- Harwit E. WeChat: Social and political development of China’s dominant messaging app. Chinese Journal of Communication 2017;10:312-27. [Crossref]
- Tong X, Wang X, Wang D, et al. Prevalence and ethnic pattern of overweight and obesity among middle-aged and elderly adults in China. Eur J Prev Cardiol 2019;26:1785-9. [Crossref] [PubMed]
- Qi S, Sun Y, Yin P, et al. Mobile Phone Use and Cognitive Impairment among Elderly Chinese: A National Cross-Sectional Survey Study. Int J Environ Res Public Health 2021;18:5695. [Crossref] [PubMed]
Cite this article as: Chen M, Peng X. The evolution and effects of mobile health (mHealth) intervention on weight management among healthy overweight/obese populations in China: a systematic review and meta-analysis. J Public Health Emerg 2022;6:29.